Load curve data visualization method based on combination of supervised and unsupervised algorithms
A technology of curve data and load curve, applied in the field of load data processing on the user side of smart grid, can solve the problems of classification result influence, low accuracy of label data, etc., to achieve the effect of optimizing internal parameters, high accuracy of data processing, and improving fitness
- Summary
- Abstract
- Description
- Claims
- Application Information
AI Technical Summary
Problems solved by technology
Method used
Image
Examples
Embodiment
[0054] Such as figure 1 Shown is the specific flow diagram of the load curve data visualization method based on the combination of supervised and unsupervised algorithms in the present invention, which specifically includes the following steps:
[0055] 1) Determine the optimal number of clusters K by sum of squared error (SSE);
[0056] 2) Select partial load curves and cluster them by bi-scale spectral clustering to obtain category label data;
[0057] 21) Distance similarity measure:
[0058] Euclidean distance is used to judge the distance similarity between curves. Euclidean distance refers to the real distance between two points in m-dimensional space. Load curve x i with y i The Euclidean distance between is defined as:
[0059]
[0060] In the formula, a i,j Indicates the Euclidean distance between two user power load curve data i and j, m represents the dimension, k, i and j are natural numbers, x i,k and x j,k Respectively represent the data values corr...
PUM
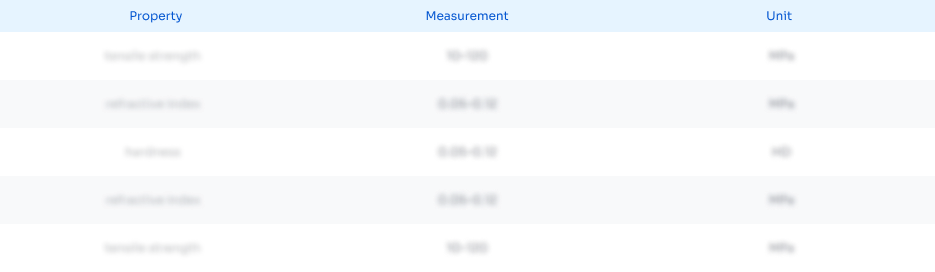
Abstract
Description
Claims
Application Information
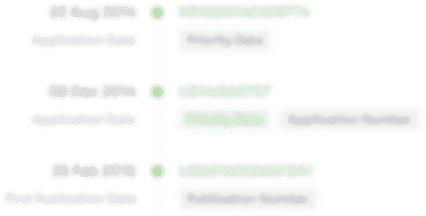
- R&D Engineer
- R&D Manager
- IP Professional
- Industry Leading Data Capabilities
- Powerful AI technology
- Patent DNA Extraction
Browse by: Latest US Patents, China's latest patents, Technical Efficacy Thesaurus, Application Domain, Technology Topic, Popular Technical Reports.
© 2024 PatSnap. All rights reserved.Legal|Privacy policy|Modern Slavery Act Transparency Statement|Sitemap|About US| Contact US: help@patsnap.com