Construction method and application of deep convolutional neural network with resolution adaptability
A deep convolution and neural network technology, applied in the field of deep convolutional neural networks, can solve the problems of wasting image information, increasing computational burden, pixel information loss, etc., to prevent loss of information, avoid computational burden, and improve detection results Effect
- Summary
- Abstract
- Description
- Claims
- Application Information
AI Technical Summary
Problems solved by technology
Method used
Image
Examples
Embodiment Construction
[0031] In order to make the technical means, creation features, achievement goals and effects of the present invention easy to understand, the following describes the deep convolutional neural network with resolution adaptability of the present invention in conjunction with the embodiments and the accompanying drawings.
[0032]
[0033] The platform implemented by this embodiment uses ubuntu16.04 for the operating system, pytorch1.01 for the deep learning framework, opencv 3.2.0 for the graphics processing library, CUDA version 9.0, and NVIDIA1080Ti GPU for the image acceleration computing unit.
[0034] In this embodiment, the Pascal VOC data set is used as the processing object, and the deep learning model needs to be trained and tested before being formed, and then applied to the actual scene. The design of deep convolutional neural networks is specific to the dataset. There is no difference between the training, testing and practical application of deep learning models ...
PUM
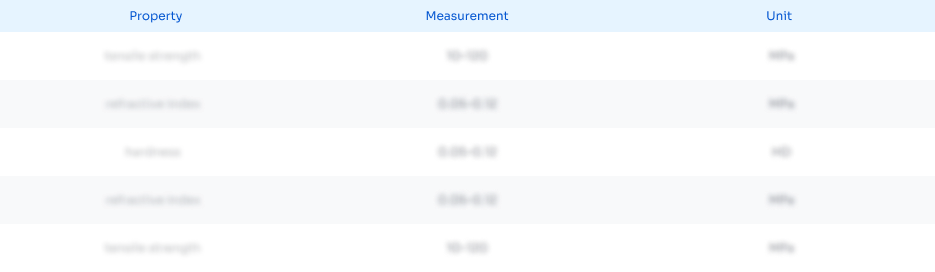
Abstract
Description
Claims
Application Information
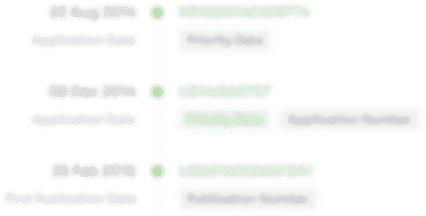
- R&D Engineer
- R&D Manager
- IP Professional
- Industry Leading Data Capabilities
- Powerful AI technology
- Patent DNA Extraction
Browse by: Latest US Patents, China's latest patents, Technical Efficacy Thesaurus, Application Domain, Technology Topic, Popular Technical Reports.
© 2024 PatSnap. All rights reserved.Legal|Privacy policy|Modern Slavery Act Transparency Statement|Sitemap|About US| Contact US: help@patsnap.com