Item active pick-up method through mechanical arm based on deep and reinforced learning
A technology of reinforcement learning and manipulators, applied in manipulators, program-controlled manipulators, manufacturing tools, etc., can solve problems such as picking failures, easy output of wrong values, and inability to solve effectively
- Summary
- Abstract
- Description
- Claims
- Application Information
AI Technical Summary
Problems solved by technology
Method used
Image
Examples
Embodiment Construction
[0071] The present invention proposes a method for actively picking up objects with a robotic arm based on deep reinforcement learning. The present invention will be further described in detail below in conjunction with the accompanying drawings and specific embodiments.
[0072] The present invention proposes a method for actively picking up objects with a robotic arm based on deep reinforcement learning. The overall process is as follows: figure 1 As shown, it specifically includes the following steps:
[0073] 1) build the simulation environment that manipulator picks up, present embodiment adopts V-REP software (Virtual RobotExperimentation Platform, virtual robot experiment platform); Concrete steps are as follows:
[0074] 1-1) Import any manipulator model that can control the movement (the manipulator model can be different from the actual manipulator) in the V-REP software as the manipulator simulation. This embodiment uses UR5 (Universal Robots 5, Universal Robots 5)...
PUM
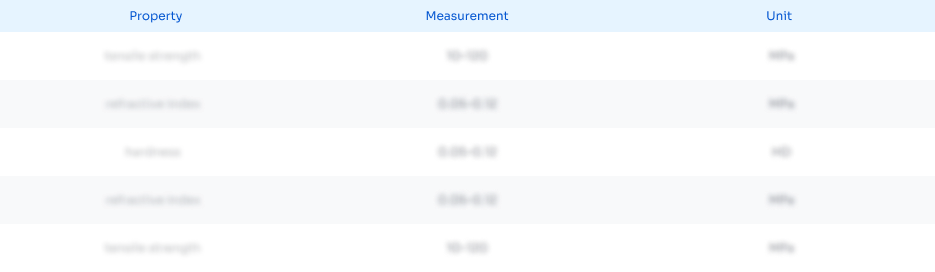
Abstract
Description
Claims
Application Information
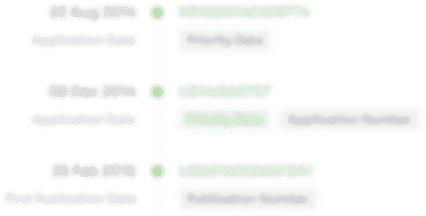
- R&D Engineer
- R&D Manager
- IP Professional
- Industry Leading Data Capabilities
- Powerful AI technology
- Patent DNA Extraction
Browse by: Latest US Patents, China's latest patents, Technical Efficacy Thesaurus, Application Domain, Technology Topic, Popular Technical Reports.
© 2024 PatSnap. All rights reserved.Legal|Privacy policy|Modern Slavery Act Transparency Statement|Sitemap|About US| Contact US: help@patsnap.com