Full-flow attack detection technology based on machine learning
An attack detection and machine learning technology, applied in machine learning, instruments, computing models, etc., can solve problems such as imperfect network supervision, sensitive data outreach, and asset destruction, so as to improve the ability of security confrontation, change working methods, and improve efficiency effect
- Summary
- Abstract
- Description
- Claims
- Application Information
AI Technical Summary
Problems solved by technology
Method used
Image
Examples
application example 1
[0033] For example, the irregular behavior analysis system can detect abnormalities in the relationship between users and servers, and the main observation object is users. Intranet traffic data, terminal log data, and database server data can be used to establish user portraits, and then machine learning algorithms can be used to calculate the association or similar relationship between users, users with similar behaviors and close associations ( image 3 The gray dots in the circles in ) can be seen as the same virtual group ( image 3 circle in ), once a user ( image 3 Some behaviors of the circles in the circle (points connected by lines), such as server login behavior, database access behavior and personal history behavior are relatively large, and at the same time, the virtual group behavior of the user is relatively large, and the user's behavior can be detected abnormal.
application example 2
[0035] For example, irregular behavior analysis can detect anomalies in host traffic, mainly observing entities. The traffic here is a general term, which can be the size of uploaded and downloaded files, the situation when the database is requested, the situation where the agent is requested, etc. As shown in the figure below, the blue curve represents the traffic change of a certain host and one service, and the red dots represent the detected abnormal points. Generally, traffic anomalies can be detected by rules or statistical models, but Figure 4 There is no significant change in the size of the medium flow itself, but the pattern of the flow that has changed, and this abnormal pattern cannot be directly described by common characteristics such as period, same frequency, and high frequency. Here, UEBA uses machine learning algorithms to automatically discover abnormalities in traffic patterns, and then trace the causes of abnormalities.
[0036] According to step (4), t...
PUM
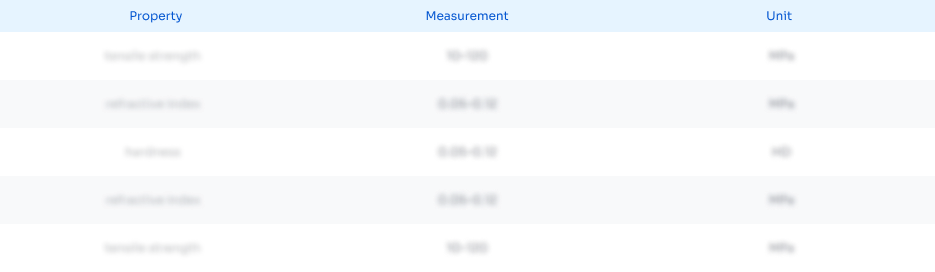
Abstract
Description
Claims
Application Information
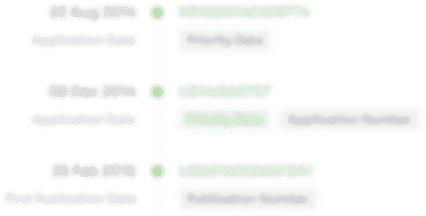
- R&D
- Intellectual Property
- Life Sciences
- Materials
- Tech Scout
- Unparalleled Data Quality
- Higher Quality Content
- 60% Fewer Hallucinations
Browse by: Latest US Patents, China's latest patents, Technical Efficacy Thesaurus, Application Domain, Technology Topic, Popular Technical Reports.
© 2025 PatSnap. All rights reserved.Legal|Privacy policy|Modern Slavery Act Transparency Statement|Sitemap|About US| Contact US: help@patsnap.com