Self-distillation training method and scalable dynamic prediction method of convolutional neural network
A technology of convolutional neural network and training method, which is applied in the field of self-distillation training method of convolutional neural network and scalable dynamic prediction, can solve problems such as hidden safety hazards, low precision, cumbersome work, etc., and achieve improved accuracy, improved performance, Effect of Accuracy Improvement
- Summary
- Abstract
- Description
- Claims
- Application Information
AI Technical Summary
Problems solved by technology
Method used
Image
Examples
Embodiment Construction
[0062] The present invention will be further described in detail below in conjunction with specific embodiments, which are explanations of the present invention rather than limitations.
[0063] Such as figure 1 As shown, the present invention proposes a self-distillation training method for convolutional neural networks, which can achieve the highest possible accuracy and overcome the shortcomings of traditional distillation when training compact models. Instead of implementing two steps in traditional distillation, i.e., the first step to train a large teacher model, and the second step to distill knowledge from the teacher model to the student model; the method of the present invention provides a one-step self-distillation framework whose training is directed to the student Model. The proposed self-distillation not only requires less training time (4.6 times shorter training time from 26.98 hours to 5.87 hours on CIFAR100), but also achieves higher accuracy (79.33 times fr...
PUM
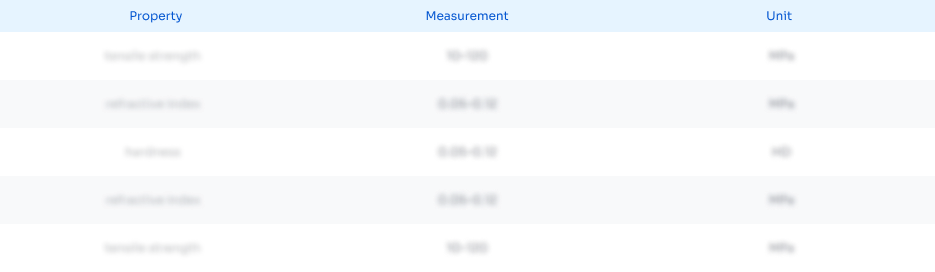
Abstract
Description
Claims
Application Information
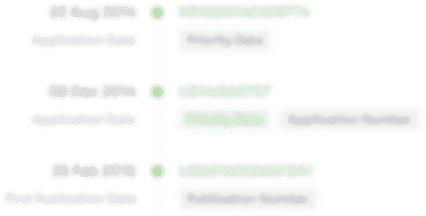
- R&D Engineer
- R&D Manager
- IP Professional
- Industry Leading Data Capabilities
- Powerful AI technology
- Patent DNA Extraction
Browse by: Latest US Patents, China's latest patents, Technical Efficacy Thesaurus, Application Domain, Technology Topic, Popular Technical Reports.
© 2024 PatSnap. All rights reserved.Legal|Privacy policy|Modern Slavery Act Transparency Statement|Sitemap|About US| Contact US: help@patsnap.com