Metal additive manufacturing forming size real-time prediction method based on deep learning
A deep learning and metal additive technology, applied in the field of additive manufacturing, can solve problems such as large error in prediction value, interference of image data, and difficulty in accurate processing, and achieve the effect of improving efficiency and accuracy
- Summary
- Abstract
- Description
- Claims
- Application Information
AI Technical Summary
Problems solved by technology
Method used
Image
Examples
Embodiment 1
[0029] Such as figure 1 As shown, the real-time prediction method of metal additive manufacturing forming size based on deep learning includes the following steps:
[0030] S1: Continuously collect molten pool images in a certain time sequence, use part of the continuous molten pool images to establish a training data set, and similarly, use part of the continuous molten pool images to establish a test data set;
[0031] S2: Establish a deep learning convolutional neural network model, set the corresponding model parameters, including the number of network layers and activation functions; the framework of the network model is Resnet101;
[0032] Such as Figure 5 As shown, the model can be divided into input module, feature extraction module, and decision-making layer module; the input module is the molten pool image, and the feature extraction module is mainly composed of convolutional layer, batch normalization layer, and activation layer. The layer module includes average...
Embodiment 2
[0048] Such as image 3 As shown, the real-time prediction system for the forming size of laser metal additive manufacturing based on deep learning includes a printing table, an image acquisition device, a human-computer interaction device, a display, and a host computer. The host is electrically connected, and the image acquisition device is installed above the printing workbench; the image acquisition device is used to continuously collect images of the molten pool in a certain time sequence and transmit the collected images of the molten pool to the Host; the host is used to set up a training data set using part of the continuous melt pool image, and similarly, also use part of the continuous melt pool image to build a test data set; set up a deep learning convolutional neural network model, and set up a corresponding model Parameters, including the number of network layers and activation functions; the framework of the network model is Resnet101, input the molten pool imag...
PUM
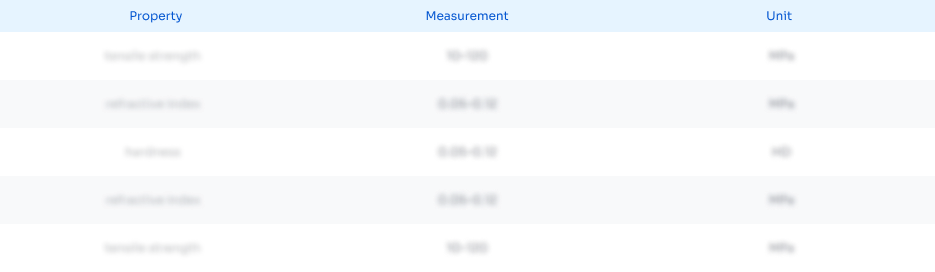
Abstract
Description
Claims
Application Information
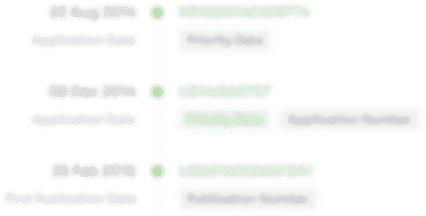
- R&D Engineer
- R&D Manager
- IP Professional
- Industry Leading Data Capabilities
- Powerful AI technology
- Patent DNA Extraction
Browse by: Latest US Patents, China's latest patents, Technical Efficacy Thesaurus, Application Domain, Technology Topic.
© 2024 PatSnap. All rights reserved.Legal|Privacy policy|Modern Slavery Act Transparency Statement|Sitemap