Low-complexity convolutional neural network based on symbol random calculation
A convolutional neural network and random computing technology, applied in the field of convolutional neural networks, can solve the problems of reducing the complexity of hardware implementation, difficulty in small wearable devices, and high consumption of convolutional neural network hardware, so as to avoid calculation errors, The effect of reducing the number and reducing the delay
- Summary
- Abstract
- Description
- Claims
- Application Information
AI Technical Summary
Problems solved by technology
Method used
Image
Examples
Embodiment Construction
[0063] The present invention will be further explained below in conjunction with the accompanying drawings and specific embodiments.
[0064] This embodiment applies the concept and technology of random computing to the convolutional neural network. First, random computing is described here. Random computing is a random 0-1 bit stream proposed by Grain in 1969. "The probability of occurrence replaces the binary value to carry out the calculation method of data processing, and its advantage lies in that it can realize complex operation function with simple logic circuit.
[0065] Such as figure 1 As shown, through our research, we found that the traditional two random computing encoding methods are not suitable for direct application to convolution computing. First of all, for Unipolar Stochastic Computing (Unipolar Stochastic Computing, USC), because the value it represents can only be a positive number, and the weight of the filter in the neural network has both positive and...
PUM
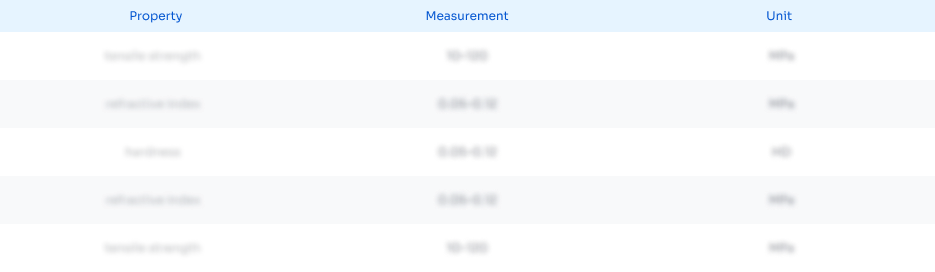
Abstract
Description
Claims
Application Information
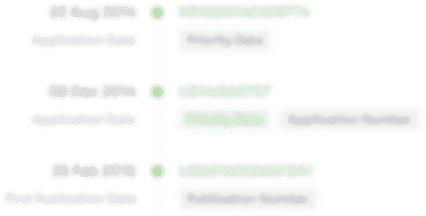
- R&D Engineer
- R&D Manager
- IP Professional
- Industry Leading Data Capabilities
- Powerful AI technology
- Patent DNA Extraction
Browse by: Latest US Patents, China's latest patents, Technical Efficacy Thesaurus, Application Domain, Technology Topic, Popular Technical Reports.
© 2024 PatSnap. All rights reserved.Legal|Privacy policy|Modern Slavery Act Transparency Statement|Sitemap|About US| Contact US: help@patsnap.com