Image feature point mismatching elimination method based on local transformation model
A technology of image feature and local transformation, applied in image analysis, image data processing, computer components, etc., can solve the problems of high mis-match rate and low accuracy of feature points, and achieve the effect of improving accuracy and accuracy
- Summary
- Abstract
- Description
- Claims
- Application Information
AI Technical Summary
Problems solved by technology
Method used
Image
Examples
specific Embodiment approach 1
[0034] Specific implementation mode 1: In this implementation mode, a method for eliminating incorrect matching of image feature points based on a local transformation model, the specific process is as follows:
[0035] Step 1. Use SIFT (Scale Invariant Feature Transform) feature point extraction algorithm to capture two images I of the same scene under different viewing angles. p and I q Detect and describe feature points;
[0036] Step 2, based on FLANN (Fast Library for Approximate Nearest Neighbors, fast nearest neighbor approximation search function library) fast nearest neighbor approximation search function library to image I p Each feature point of the image I q The feature points are searched intensively to get the image I p Each feature point in image I q The feature points of the nearest neighbor and the second nearest neighbor;
[0037] When image I p The feature points in image I q A times the distance of the nearest neighbor feature point in the image I p T...
specific Embodiment approach 2
[0050] Specific embodiment 2: The difference between this embodiment and specific embodiment 1 is that in the second step, the A times is 1.2 times.
[0051] Other steps and parameters are the same as those in Embodiment 1.
specific Embodiment approach 3
[0052] Specific embodiment three: the difference between this embodiment and specific embodiment one or two is that the RANSAC algorithm is used in the step three to classify and initially screen the set of initial feature points obtained in the step two; the specific process is:
[0053] Step 31. Set the remaining point pair set after preliminary screening as S′, and initialize it to the initial feature point pair set S obtained in step 2, and initialize the number of categories n to 0;
[0054] Step 32: Use the RANSAC (random sample consensus) algorithm to extract interior points from the point pair set S′, and the interior point feature point pair set is s n+1 , and update S′ to exclude s n+1 The set of remaining point pairs after
[0055] Step 3 and specific implementation mode 2 are a process of cyclically screening point pairs, each time step 3 and 2 are performed to extract some internal points, namely s n+1 , if the number of interior points is greater than or equal ...
PUM
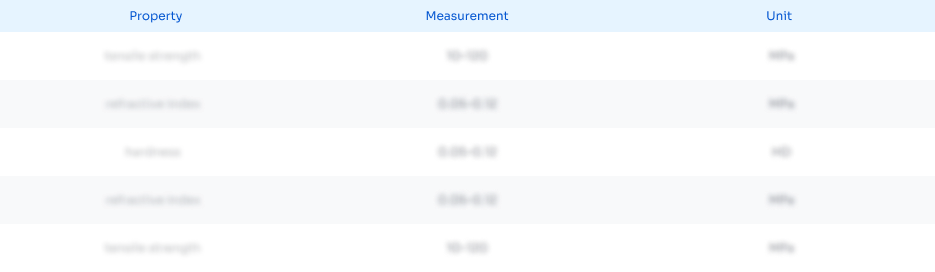
Abstract
Description
Claims
Application Information
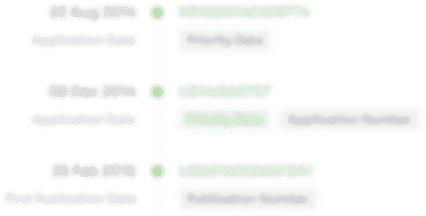
- R&D
- Intellectual Property
- Life Sciences
- Materials
- Tech Scout
- Unparalleled Data Quality
- Higher Quality Content
- 60% Fewer Hallucinations
Browse by: Latest US Patents, China's latest patents, Technical Efficacy Thesaurus, Application Domain, Technology Topic, Popular Technical Reports.
© 2025 PatSnap. All rights reserved.Legal|Privacy policy|Modern Slavery Act Transparency Statement|Sitemap|About US| Contact US: help@patsnap.com