Rolling bearing residual life prediction method based on deep generative adversarial network
A technology for rolling bearings and life prediction, applied in biological neural network models, neural learning methods, character and pattern recognition, etc., can solve problems such as limiting the application of data-driven methods, unresolved, and failures
- Summary
- Abstract
- Description
- Claims
- Application Information
AI Technical Summary
Problems solved by technology
Method used
Image
Examples
Embodiment Construction
[0076] The present invention will be further described below in conjunction with the accompanying drawings and embodiments.
[0077] Such as figure 1 As shown, a method for predicting the remaining life of rolling bearings based on deep generative adversarial networks includes the following steps:
[0078] 1) Collect the original vibration signal of the rolling bearing.
[0079] The original vibration signal of the rolling bearing includes two kinds of horizontal vibration signal and vertical vibration signal, which are respectively measured by the accelerometer.
[0080] 2) Obtain the characteristic parameters of the original vibration signal.
[0081] The original vibration signal is preprocessed to obtain characteristic parameters, which include root mean square value, standard deviation, peak value and average value.
[0082] 3) Divide the feature parameters obtained in step 2) into a training set and a prediction set.
[0083] Consider the statistical feature paramete...
PUM
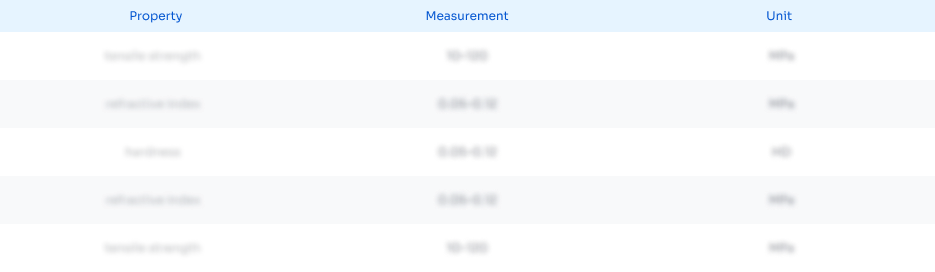
Abstract
Description
Claims
Application Information
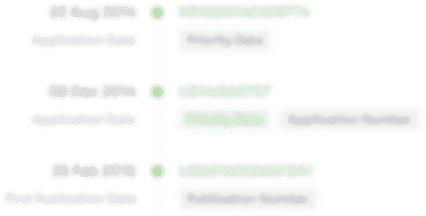
- R&D Engineer
- R&D Manager
- IP Professional
- Industry Leading Data Capabilities
- Powerful AI technology
- Patent DNA Extraction
Browse by: Latest US Patents, China's latest patents, Technical Efficacy Thesaurus, Application Domain, Technology Topic, Popular Technical Reports.
© 2024 PatSnap. All rights reserved.Legal|Privacy policy|Modern Slavery Act Transparency Statement|Sitemap|About US| Contact US: help@patsnap.com