Natural gas pipeline leakage detection method based on LSTM recurrent neural network
A cyclic neural network, natural gas pipeline technology, applied in pipeline systems, gas/liquid distribution and storage, mechanical equipment, etc., can solve problems such as corrosion, time difference, accurate real-time detection of pipeline leakage, etc., and achieve broad and sufficient theoretical research value. Response time, the effect of improving accuracy
- Summary
- Abstract
- Description
- Claims
- Application Information
AI Technical Summary
Problems solved by technology
Method used
Image
Examples
Embodiment Construction
[0044] In order to make the object, technical solution and advantages of the present invention more clear, the present invention will be further described in detail below in conjunction with the examples. It should be understood that the specific embodiments described here are only used to explain the present invention, not to limit the present invention.
[0045] The meaning of the Chinese and English abbreviations of the present invention is illustrated below:
[0046] LSTM: Long-short-term memory network, which can effectively solve the problem of poor stability and large fluctuations of audio signals in the CNN neural network, and can also solve the problem of long-distance dependence that RNN cannot handle.
[0047] MFCC: Mel Frequency Cepstral Coefficients.
[0048] LMD: local mean decomposition.
[0049] Fbank: filter bank.
[0050] DCT: Performs a discrete cosine transform.
[0051] LMFCC: Improved MFCC.
[0052] ΔLMFCC: Improved first-order difference of MFCC.
...
PUM
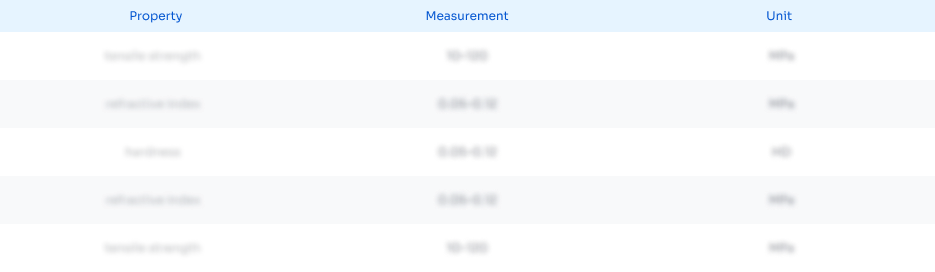
Abstract
Description
Claims
Application Information
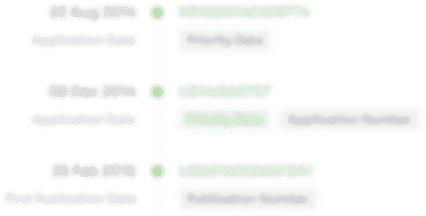
- R&D
- Intellectual Property
- Life Sciences
- Materials
- Tech Scout
- Unparalleled Data Quality
- Higher Quality Content
- 60% Fewer Hallucinations
Browse by: Latest US Patents, China's latest patents, Technical Efficacy Thesaurus, Application Domain, Technology Topic, Popular Technical Reports.
© 2025 PatSnap. All rights reserved.Legal|Privacy policy|Modern Slavery Act Transparency Statement|Sitemap|About US| Contact US: help@patsnap.com