5G energy internet virtual power plant economic dispatching method based on edge intelligence
An energy Internet and virtual power plant technology, which is applied in the field of 5G energy Internet virtual power plant economic dispatch based on edge intelligence to achieve the effects of reducing costs, reducing consumption and reducing demand
- Summary
- Abstract
- Description
- Claims
- Application Information
AI Technical Summary
Problems solved by technology
Method used
Image
Examples
specific Embodiment approach 1
[0026] Specific implementation mode 1: An edge intelligence-based 5G energy Internet virtual power plant economic scheduling method described in this implementation mode, the method includes the following steps:
[0027] Step 1. Construct an Actor-Critic architecture including actor target network, actor estimation network, critic target network and critic estimation network;
[0028] Step 2. Input the state s of the virtual power plant into the actor estimation network;
[0029] Step 3: Use deterministic policy gradient to select the action a corresponding to the state s, and then use the state s and action a to calculate the reward function R and the next state s′, and obtain an experience fragment (s, a, R, s′ ), and store the obtained experience fragments (s, a, R, s′) into the experience pool;
[0030] Step 4. Input the state s' into the actor estimation network;
[0031] Step 5. Repeat the process of step 3 and step 4 until the termination state is reached to complete ...
specific Embodiment approach 2
[0055] Specific implementation mode two: the difference between this implementation mode and specific implementation mode one is:
[0056] The actor target network is a deep neural network composed of 3 hidden layers fully connected with 10 neural units per layer, and the structure of the actor estimation network is the same as that of the actor target network;
[0057] The critic target network is a deep neural network composed of 5 hidden layers fully connected with 20 neurons in each layer, and the structure of the critic estimation network is the same as that of the critic target network.
specific Embodiment approach 3
[0058] Specific embodiment three: the difference between this embodiment and specific embodiment one is: the neuron activation functions of the hidden layer of the actor target network, actor estimation network, critic target network, and critic estimation network are all relu functions, and the neuron activation functions of the output layer The activation functions are all linear activation functions.
[0059] The weight is initialized to mean 0, the covariance matrix is 1, the PC used in the experiment is 4-core CPU Inter(R)core i7-5770, 8GB of RAM and GPU is RTX 1060, 5G RAM machine, python is 1.36.0 Version.
[0060] For the Critic estimation network, its loss function is the mean square error of Q estimation and Q reality, namely:
[0061]
[0062] For the actor estimation network, its loss function is different from that of DPG, because it is a deep deterministic strategy. Then the defined loss gradient is:
[0063]
[0064] Actor estimation network loss func...
PUM
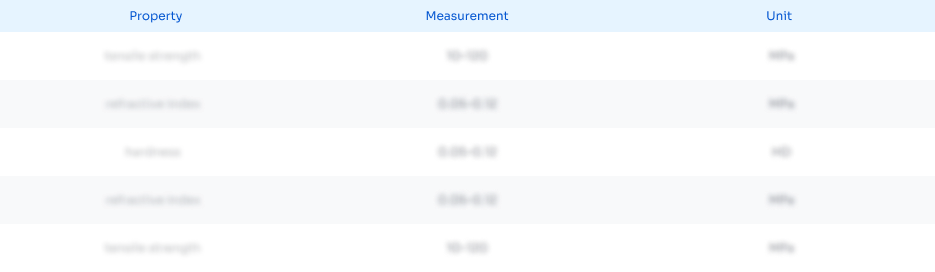
Abstract
Description
Claims
Application Information
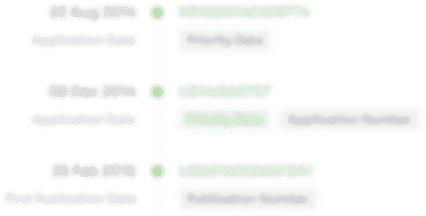
- R&D
- Intellectual Property
- Life Sciences
- Materials
- Tech Scout
- Unparalleled Data Quality
- Higher Quality Content
- 60% Fewer Hallucinations
Browse by: Latest US Patents, China's latest patents, Technical Efficacy Thesaurus, Application Domain, Technology Topic, Popular Technical Reports.
© 2025 PatSnap. All rights reserved.Legal|Privacy policy|Modern Slavery Act Transparency Statement|Sitemap|About US| Contact US: help@patsnap.com