Fatigue factor recessive anomaly detection and fault diagnosis method based on LSTM
A fault diagnosis and anomaly detection technology, applied in the direction of instruments, biological neural network models, character and pattern recognition, etc., can solve problems such as equipment data imbalance and high noise
- Summary
- Abstract
- Description
- Claims
- Application Information
AI Technical Summary
Problems solved by technology
Method used
Image
Examples
Embodiment Construction
[0066] The present invention will be further described in detail below in conjunction with the accompanying drawings and specific embodiments.
[0067] S1. Collect the time-series sample data of the target equipment for diagnosis. The sample contains normal data, abnormal data and the fault type of the abnormal data. Use the EMD method to perform empirical mode decomposition on the time-series sample data of the equipment;
[0068] The experimental verification of the present invention uses experimental data including three types of faults: inner raceway fault, outer raceway fault and ball fault;
[0069] S2. Extract the normal data samples in the time series sample data, and use the deep neural network based on LSTM to construct the equipment vibration signal prediction model;
[0070] The process flow of the LSTM-based vibration signal prediction model proposed by the present invention is described as follows, and the model flow chart is shown in Figure 1.
[0071] Step 1: ...
PUM
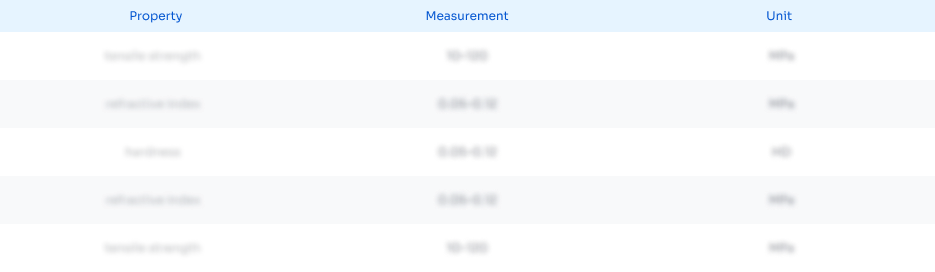
Abstract
Description
Claims
Application Information
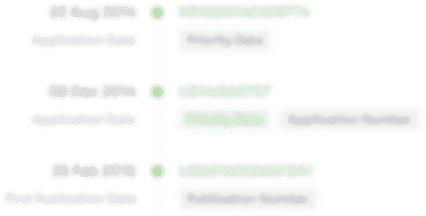
- R&D Engineer
- R&D Manager
- IP Professional
- Industry Leading Data Capabilities
- Powerful AI technology
- Patent DNA Extraction
Browse by: Latest US Patents, China's latest patents, Technical Efficacy Thesaurus, Application Domain, Technology Topic.
© 2024 PatSnap. All rights reserved.Legal|Privacy policy|Modern Slavery Act Transparency Statement|Sitemap