Part surface roughness and tool wear prediction method based on multi-task learning
A surface roughness, multi-task learning technology, applied in the field of machining, to reduce costs, avoid repetitive work, and improve production efficiency and quality
- Summary
- Abstract
- Description
- Claims
- Application Information
AI Technical Summary
Problems solved by technology
Method used
Image
Examples
Embodiment Construction
[0049] In order to make the purpose, technical solution and advantages of the present invention clearer, the present invention will be described in detail below in conjunction with the accompanying drawings.
[0050] On a three-axis vertical machining center, a cutting test was performed with a vertical milling cutter. Among them, the basic information of the three-axis vertical machining center is: the maximum travel of the X-axis, Y-axis and Z-axis is 710mm, 500mm and 350mm, and the maximum feed speed is 32m / min, 32m / min and 30m / min; The highest speed is 15000r / min. The basic information of the tool is: the tool type is vertical milling cutter; the tool material is carbide; the tool diameter is 10mm; the number of tool edges is 4. The basic information of the workpiece to be cut is: the material of the workpiece is 45# steel; the shape of the workpiece is 200mm X 100mm X 10mm. The cutting process parameters are: depth of cut is 2mm; feed rate is 80mm / min; spindle speed is ...
PUM
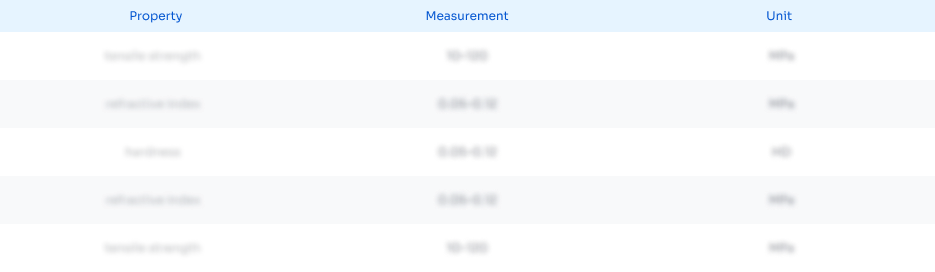
Abstract
Description
Claims
Application Information
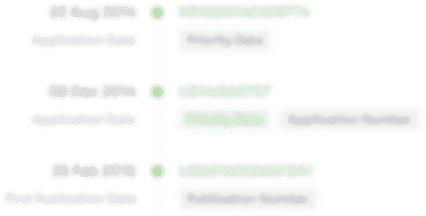
- R&D Engineer
- R&D Manager
- IP Professional
- Industry Leading Data Capabilities
- Powerful AI technology
- Patent DNA Extraction
Browse by: Latest US Patents, China's latest patents, Technical Efficacy Thesaurus, Application Domain, Technology Topic, Popular Technical Reports.
© 2024 PatSnap. All rights reserved.Legal|Privacy policy|Modern Slavery Act Transparency Statement|Sitemap|About US| Contact US: help@patsnap.com