Multi-view data missing completion method for multi-manifold regularization non-negative matrix factorization
A technique for non-negative matrix factorization and missing data, applied in the field of machine learning
- Summary
- Abstract
- Description
- Claims
- Application Information
AI Technical Summary
Problems solved by technology
Method used
Image
Examples
Embodiment Construction
[0069] The invention provides a multi-view data missing complement method based on multi-manifold regularized non-negative matrix decomposition, which does not require large-scale labeled samples for training, not only avoids pre-defining category relationships and related features, but also improves existing Multi-view mining technology has the ability to understand and discover unlabeled multi-source data; it also solves the estimation bias and statistical power loss caused by the deletion method in the traditional missing processing method, and reduces the sample distribution distortion that may be caused by the single imputation method ; It provides a new method for accurate completion of multi-view and multi-attribute missing data in an unsupervised environment.
[0070] see figure 1 , the present invention is based on multi-manifold regularized non-negative matrix decomposition multi-view data missing complement method, comprising the following steps:
[0071] S1. Throu...
PUM
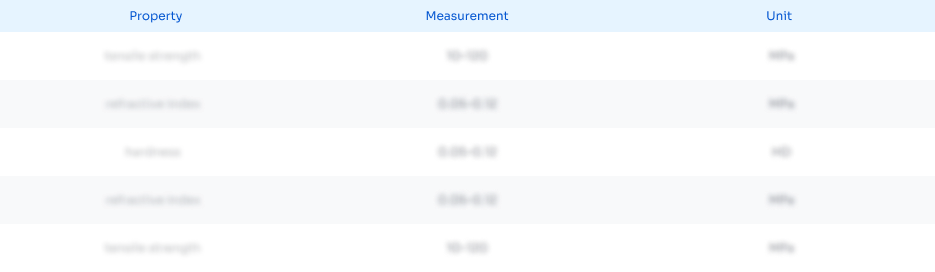
Abstract
Description
Claims
Application Information
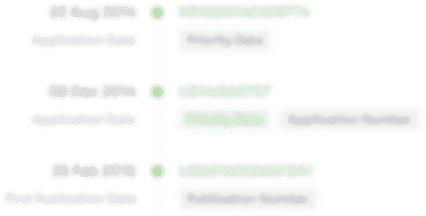
- Generate Ideas
- Intellectual Property
- Life Sciences
- Materials
- Tech Scout
- Unparalleled Data Quality
- Higher Quality Content
- 60% Fewer Hallucinations
Browse by: Latest US Patents, China's latest patents, Technical Efficacy Thesaurus, Application Domain, Technology Topic, Popular Technical Reports.
© 2025 PatSnap. All rights reserved.Legal|Privacy policy|Modern Slavery Act Transparency Statement|Sitemap|About US| Contact US: help@patsnap.com