Using convolution neural networks for on-the-fly single particle reconstruction
A neural network and artificial neural network technology, applied in the field of particle selection, can solve problems such as difficulty in developing, identifying and classifying different particles
- Summary
- Abstract
- Description
- Claims
- Application Information
AI Technical Summary
Problems solved by technology
Method used
Image
Examples
example 1
[0026] refer to figure 1 , a representative system 100 for picking up particles for structure determination or other applications includes an imaging system 102, typically a transmission electron microscope (TEM), which provides an image of a particle-laden substrate to Image processor 104 to identify expected types of particles and reject undesired particles. A TEM or other electron microscope generally includes an electron beam source, electron optics, and an electron detection system that captures or produces electron beam-based images, also referred to herein as an electron beam column. Particle identification can be challenging due to the generally limited quality of images produced by the imaging system 102 . The image processor 104 may be implemented in the form of a processor associated with the imaging system 102 or associated with a separate local processing device as discussed, or associated with a remote processor coupled by a local or wide area network 106 to Th...
example 2
[0029] refer to figure 2 , a representative workflow 200 for particle picking includes providing a TEM grid 202 on which selected particles are distributed. In some examples, TEM grid 202 is a so-called "porous" carbon-coated TEM support or foil that contains a plurality of pores. Particles may be retained in the ice layer at some or all of the holes on grid 202 or at other locations. One or more images (also referred to as "micrographs") of a network region are obtained at 204, and specific mesh regions are selected at 206. Such as figure 2 As shown, image portions 208 , 210 correspond to holes in network 202 . At 212 a final portion of an image or a corresponding portion of a series of images is selected. In some cases, photomicrographs were not selected due to significant defocus; substantial ice contamination; or images containing only carbon support and no pore regions. With a perfect particle picking method, manual selection of photomicrographs may not be necessar...
example 3
[0031] refer to image 3 , method 300 of selecting particles of a particular type includes exposing one or more particle samples to an electron beam at 302 and obtaining an associated image at 304 . At 306, a contrast transfer function correction is applied to the images, and image frames from the series of images are aligned and combined. At 308, some images (micrographs) are selected for further processing. At 310, multiple CNNs are applied to selected images to pick and classify particle images and indicate particle alignment. At 312, an average particle image of common types and arrangements of particles can be displayed. At 314, a final particle set of preferred particle types is selected, and at 316, some or all of the selected particle images may be used to evaluate particle structure.
[0032] After the final set of particles is selected at 314, the selected set of particles may be used to refine the neural network for particle selection, or to train or refine anoth...
PUM
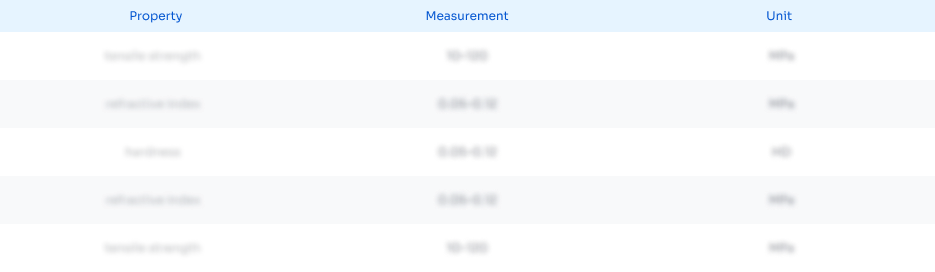
Abstract
Description
Claims
Application Information
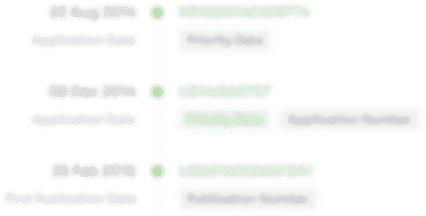
- R&D Engineer
- R&D Manager
- IP Professional
- Industry Leading Data Capabilities
- Powerful AI technology
- Patent DNA Extraction
Browse by: Latest US Patents, China's latest patents, Technical Efficacy Thesaurus, Application Domain, Technology Topic.
© 2024 PatSnap. All rights reserved.Legal|Privacy policy|Modern Slavery Act Transparency Statement|Sitemap