Lithium battery health state estimation method based on convolutional neural network and transfer learning
A convolutional neural network and health status technology, applied in the field of automation, can solve the problem of time-consuming data collection
- Summary
- Abstract
- Description
- Claims
- Application Information
AI Technical Summary
Problems solved by technology
Method used
Image
Examples
Embodiment
[0137] In this embodiment, the specific steps are as follows:
[0138] In step (1), the input data of the convolutional neural network is obtained.
[0139] a. Select 3 brand-new SONYUS18650VTC6 lithium batteries and 3 brand-new FST2000 lithium batteries, and conduct a complete overcharge, over-discharge aging test and 75 normal speed aging cycles for each model. Select 1 SONYUS18650VTC6 model and 1 FST2000 model waste battery near the end of life, and carry out the normal speed aging test respectively, and consume the battery capacity according to the cycle of constant current charging, constant voltage charging and constant current discharging until the healthy state drops to 80% % or less, about 35 to 40 cycles are sufficient. A total of 8 sets of data were obtained above. The upper and lower limits of cut-off voltage for normal aging are 4.2V and 2.75V respectively, the upper limit of overcharge cut-off voltage is 4.4V, and the lower limit of over-discharge cut-off volta...
PUM
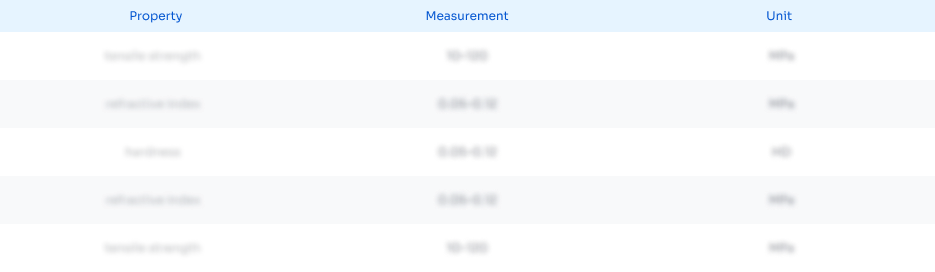
Abstract
Description
Claims
Application Information
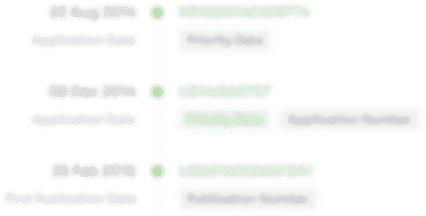
- R&D Engineer
- R&D Manager
- IP Professional
- Industry Leading Data Capabilities
- Powerful AI technology
- Patent DNA Extraction
Browse by: Latest US Patents, China's latest patents, Technical Efficacy Thesaurus, Application Domain, Technology Topic, Popular Technical Reports.
© 2024 PatSnap. All rights reserved.Legal|Privacy policy|Modern Slavery Act Transparency Statement|Sitemap|About US| Contact US: help@patsnap.com