Process manufacturing industry irregular sampling dynamic sequence modeling method based on sampling interval perception long and short term memory network
A technology of long short-term memory and sampling interval, applied in biological neural network models, manufacturing computing systems, neural learning methods, etc., which can solve problems such as difficulty for operators to maintain sampling frequency, irregular time length, sampling measurement, etc.
- Summary
- Abstract
- Description
- Claims
- Application Information
AI Technical Summary
Problems solved by technology
Method used
Image
Examples
Embodiment 1
[0130] see Figure 1-5 As shown, a method for modeling irregular sampling dynamic sequences in the process manufacturing industry based on sampling interval-aware long-term short-term memory network includes the following steps:
[0131] Step (1), selecting 43 variables (as shown in Table 1) that have an impact on the C5 content of light naphtha at the initial boiling point of jet fuel from the hydrocracking process as input variables, extracted from September 15, 2016 1,300 samples obtained from offline testing from March 1st to February 9th, 2018.
[0132] Step (2), normalize the data collected in step (1) to obtain a new data set, the conversion function is:
[0133]
[0134] where x min is the minimum value of the data set, x max is the maximum value of the dataset. Standardization of deviation is a linear transformation of the original data, so that the result falls into the [0,1] interval;
[0135] The first 1000 samples are used as the training set to train the ...
Embodiment 2
[0175] The final boiling point of heavy naphtha is predicted as follows:
[0176] Step (1), select 43 variables (as shown in Table 1) that affect the end boiling point of heavy naphtha from the hydrocracking process as input variables, and extract the data from September 15, 2016 to November 2018 871 samples obtained from offline testing on the 30th.
[0177] Step (2), normalize the data collected in step (1) to obtain a new data set, the conversion function is:
[0178]
[0179] where x min is the minimum value of the data set, x max is the maximum value of the dataset. Standardization of deviation is a linear transformation of the original data, so that the result falls into the [0,1] interval;
[0180] The first 632 samples are used as the training set to train the model parameters, and the remaining 239 samples are used as the test set to test the predictive performance of the model. First, the input and output matrices of the training set are obtained:
[0181] ...
PUM
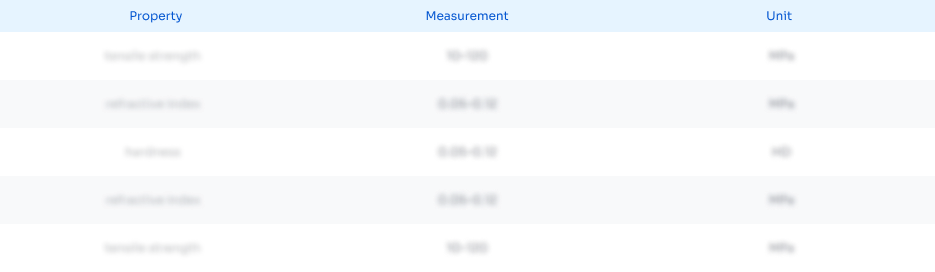
Abstract
Description
Claims
Application Information
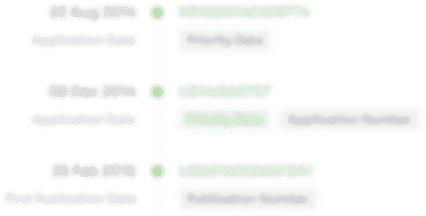
- R&D
- Intellectual Property
- Life Sciences
- Materials
- Tech Scout
- Unparalleled Data Quality
- Higher Quality Content
- 60% Fewer Hallucinations
Browse by: Latest US Patents, China's latest patents, Technical Efficacy Thesaurus, Application Domain, Technology Topic, Popular Technical Reports.
© 2025 PatSnap. All rights reserved.Legal|Privacy policy|Modern Slavery Act Transparency Statement|Sitemap|About US| Contact US: help@patsnap.com