Power load prediction method and device
A technology of power load and forecasting method, which is applied in the field of power load forecasting methods and devices, and can solve problems such as large time and resources, low forecasting accuracy and authenticity, and costly power load forecasting methods, so as to avoid data loss and waste Effects of time and resources
- Summary
- Abstract
- Description
- Claims
- Application Information
AI Technical Summary
Problems solved by technology
Method used
Image
Examples
Embodiment 1
[0085] Such as figure 1 Shown, a kind of electric load forecasting method, described method comprises:
[0086] Step S1: Input the original data set into the BP neural network, traverse and search to obtain the location of the missing data, use the BP neural network to use the load data at three time points before and after the missing data point as input features, output the missing data value, and fill in the missing data. Obtain a complete data set; among them, the original data set is a data set directly obtained from the data source without any processing, which includes weather data for a period of time: temperature, humidity, wind speed, and corresponding power load data.
[0087] Such as figure 2 As shown, the step S1 includes:
[0088] Step 101: Construct a BP neural network, wherein the input layer is 6 neurons, the middle layer is 13 neurons, the output layer is 1 neuron, and the activation function uses the sigmoid function;
[0089] Step 102: search and read a...
Embodiment 2
[0126] Corresponding to Embodiment 1 of the present invention, Embodiment 2 of the present invention also provides a power load forecasting device, which includes:
[0127] The missing data processing module is used to input the original data set into the BP neural network, traverse and search to obtain the position of the missing data, use the BP neural network to use the load data of three time points before and after the missing data point as the input feature, and output the missing data value, and carry out Fill in missing data to obtain a complete data set;
[0128] The parameter optimization module is used to optimize the parameters of the complete data set, and output the optimal hyperparameter to generate the optimal hyperparameter vector;
[0129] The model training module is used to construct the LSTM neural network using the optimal hyperparameter vector, and input the training data to train the LSTM neural network to obtain the LSTM model;
[0130] The test modul...
PUM
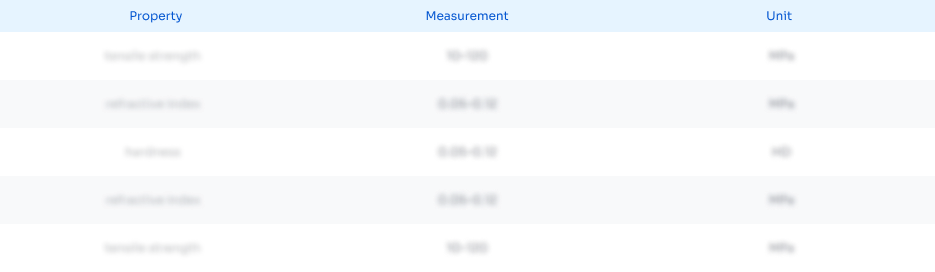
Abstract
Description
Claims
Application Information
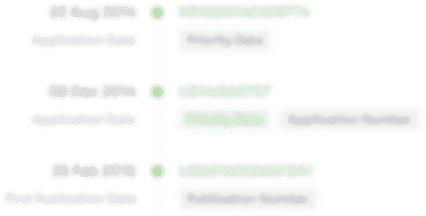
- Generate Ideas
- Intellectual Property
- Life Sciences
- Materials
- Tech Scout
- Unparalleled Data Quality
- Higher Quality Content
- 60% Fewer Hallucinations
Browse by: Latest US Patents, China's latest patents, Technical Efficacy Thesaurus, Application Domain, Technology Topic, Popular Technical Reports.
© 2025 PatSnap. All rights reserved.Legal|Privacy policy|Modern Slavery Act Transparency Statement|Sitemap|About US| Contact US: help@patsnap.com