Flood disaster prediction and early warning method based on QR-BC-ELM
A QR-BC-ELM, flood disaster technology, applied in neural learning methods, biological neural network models, instruments, etc., can solve the problems of complex calculation, low prediction accuracy, and one-sided feature response.
- Summary
- Abstract
- Description
- Claims
- Application Information
AI Technical Summary
Problems solved by technology
Method used
Image
Examples
Embodiment 1
[0054] refer to figure 2 , the multi-factor index in the step S1 includes a total of 49 calculated indexes in two criterion layers and three index layers.
Embodiment 2
[0056] The risk evaluation system evaluation step of step S2 includes:
[0057] S201: Collect the maximum three-day precipitation (M3PD), topographic wetting index (TWI), river power index (SPI) and normalized difference vegetation index (NDVI) from different hydrological stations as input to the model, and obtain different The degree of correlation between hydrological stations;
[0058] S202: Predict the water level change of the dependent variable observation station according to the index change of the independent variable observation station;
[0059] S203: For non-time series data, the digital elevation model (DEM), soil texture index (ST), slope index (SL) and land use pattern (LUP) of each variable site are used as input to the model, and the same indicators of the dependent site are used as output , to calculate the index features of highly correlated points;
[0060] S204: Taking distance index (DR), river power index (SPI) and socio-economic index as input, statis...
Embodiment 3
[0063] In the traditional extreme learning machine, the feature engineering of each indicator is advanced, and input to the extreme learning machine model in the form of time series data as the input matrix of the model, and the runoff is used as the output to correct the weight by means of reverse error propagation, so that Get the contribution of each indicator to the flood. In the past, when solving the least squares solution, the singular value decomposition is usually used to solve the matrix H, and then its plus sign generalized inverse is solved, and then the optimal least squares solution is solved as the training weight. Based on the above analysis, the core of extreme learning part is to H + operation.
[0064] This application extracts the feature engineering of multi-factor indicators and inputs them into the improved extreme learning model in the form of time series data to calculate the contribution of each indicator to the flood.
[0065] (1) The calculation p...
PUM
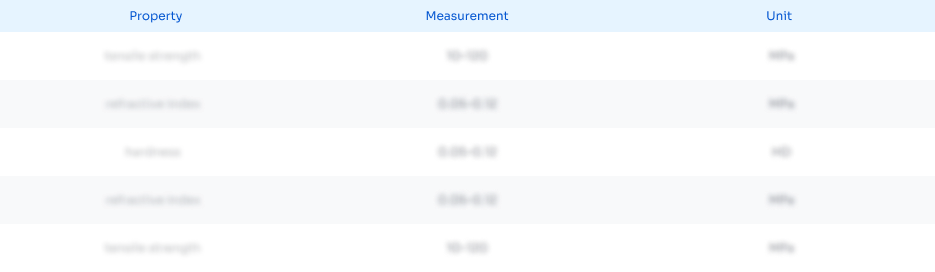
Abstract
Description
Claims
Application Information
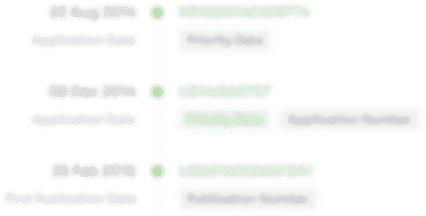
- R&D
- Intellectual Property
- Life Sciences
- Materials
- Tech Scout
- Unparalleled Data Quality
- Higher Quality Content
- 60% Fewer Hallucinations
Browse by: Latest US Patents, China's latest patents, Technical Efficacy Thesaurus, Application Domain, Technology Topic, Popular Technical Reports.
© 2025 PatSnap. All rights reserved.Legal|Privacy policy|Modern Slavery Act Transparency Statement|Sitemap|About US| Contact US: help@patsnap.com