Rumor detection method combining self-attention mechanism and generative adversarial network
A detection method and attention technology, applied in the field of text recognition, can solve problems such as the unsatisfactory effect of extracting text semantics and key features, reduce the model's ability to extract key features, and the sequence model cannot solve the problem of feature extraction, so as to avoid rumor samples. The effect of large demand, reduction of time complexity, and enhancement of semantic feature recognition ability
- Summary
- Abstract
- Description
- Claims
- Application Information
AI Technical Summary
Problems solved by technology
Method used
Image
Examples
Embodiment Construction
[0025] use figure 1 The rumor detection model shown, the rumor detection method combining the self-attention mechanism and the generation confrontation network, includes the following steps in sequence:
[0026] Step 1: Collect rumor text data to form a rumor data set; preprocess the text data, filter the text data with special symbols, and quantize words;
[0027] Step 2: Build a generative adversarial network generator including a self-attention layer, encode and decode the original text, such as figure 2 As shown, the feature distribution is biased towards the opposite category as much as possible, and the difference between the original sequence and the fake sequence is increased as much as possible, so as to strengthen the rumor detection ability of the discriminator model;
[0028] Step 3: Build a GAN discriminator, such as image 3 As shown, the rumor detection is performed on the original text and the text sequence decoded by the generator, and the same label text i...
PUM
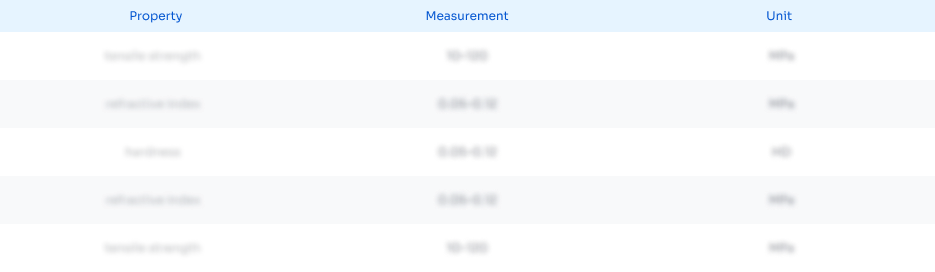
Abstract
Description
Claims
Application Information
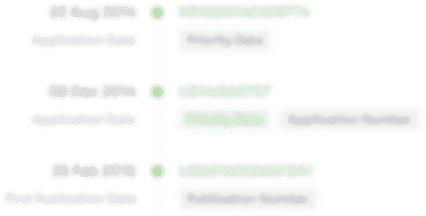
- R&D Engineer
- R&D Manager
- IP Professional
- Industry Leading Data Capabilities
- Powerful AI technology
- Patent DNA Extraction
Browse by: Latest US Patents, China's latest patents, Technical Efficacy Thesaurus, Application Domain, Technology Topic, Popular Technical Reports.
© 2024 PatSnap. All rights reserved.Legal|Privacy policy|Modern Slavery Act Transparency Statement|Sitemap|About US| Contact US: help@patsnap.com