Multivariable clustering and fusion time series combination prediction method
A time series and combined forecasting technology, applied in neural learning methods, instruments, biological neural network models, etc., can solve the problems of neural network models without a learning mechanism, insufficient data structure feature information mining, etc., and achieve strong robustness. Effect
- Summary
- Abstract
- Description
- Claims
- Application Information
AI Technical Summary
Problems solved by technology
Method used
Image
Examples
Embodiment
[0090] The example selects 37 variables (the 38th variable is the Gaussian noise introduced) related to the service of the main equipment of the compressor unit in Table 2, including equipment and process variables. The 12-day monitoring data during normal operation of the unit and the 1-day monitoring data during the fault state are selected for subsequent complex network modeling and time series prediction of target monitoring variables, and the sampling interval is 1 min.
[0091] Table 2 Main monitoring points and descriptions
[0092]
[0093]
[0094] Step 1: Analysis of coupled Granger causality measures for monitoring time series
[0095] Based on the CGC coupled Granger causality measure analysis, the coupling causality between the monitoring time series is calculated, and the Gaussian noise sequence is introduced as a comparison of the coupling causality coefficient, as the lower threshold of the coupling causality coefficient, and the coupling causality coeffi...
PUM
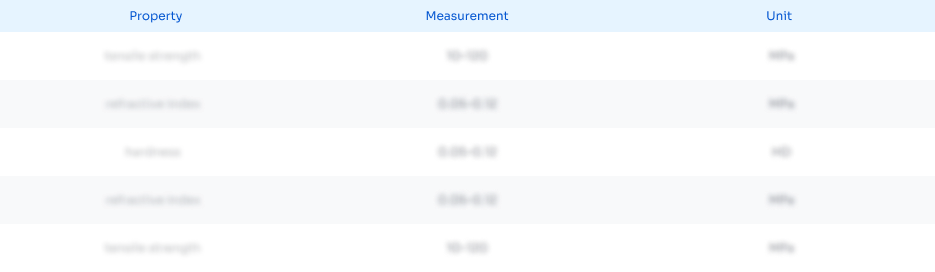
Abstract
Description
Claims
Application Information
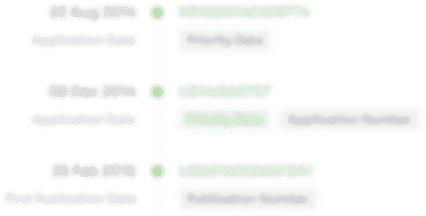
- R&D Engineer
- R&D Manager
- IP Professional
- Industry Leading Data Capabilities
- Powerful AI technology
- Patent DNA Extraction
Browse by: Latest US Patents, China's latest patents, Technical Efficacy Thesaurus, Application Domain, Technology Topic, Popular Technical Reports.
© 2024 PatSnap. All rights reserved.Legal|Privacy policy|Modern Slavery Act Transparency Statement|Sitemap|About US| Contact US: help@patsnap.com