Building energy consumption prediction method based on long-term and short-term memory network hybrid model
A technology of long-term and short-term memory and building energy consumption, which can be applied to biological neural network models, predictions, neural learning methods, etc., and can solve problems such as accuracy errors
- Summary
- Abstract
- Description
- Claims
- Application Information
AI Technical Summary
Problems solved by technology
Method used
Image
Examples
Embodiment Construction
[0074] The specific embodiments of the present invention will be described below with reference to the accompanying drawings, so that those skilled in the art can better understand the present invention.
[0075] figure 1 Unroll the topology map for Simple Recurrent Neural Networks - Real Time.
[0076] Generally, neural network models can be divided into feedforward neural network (FFNN) and recurrent neural network (RNN). FFNNs are widely used to process data in the spatial domain while ignoring data occurrences about time (i.e., temporal information). On the other hand, RNN architectures can be viewed as loop-back architectures with interconnected neurons that can model sequential and temporal dependencies between data on a larger scale [43] .
[0077] The standard for RNN architecture is in figure 1 given in. Each node in the network starts from the current state (x t ) receives input and returns from the previous state (h (t-1) ) receives the hidden state value of ...
PUM
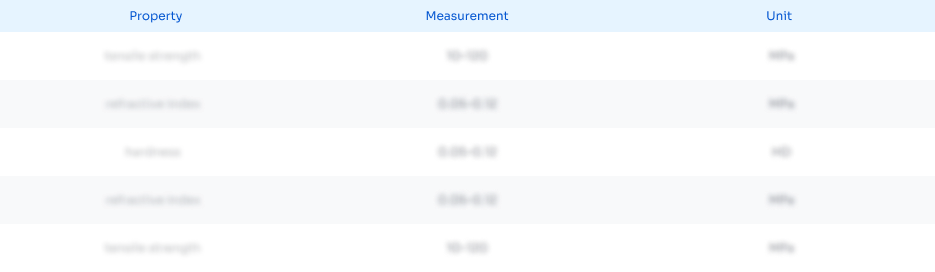
Abstract
Description
Claims
Application Information
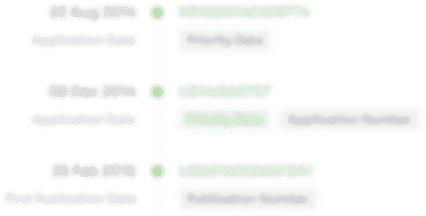
- R&D Engineer
- R&D Manager
- IP Professional
- Industry Leading Data Capabilities
- Powerful AI technology
- Patent DNA Extraction
Browse by: Latest US Patents, China's latest patents, Technical Efficacy Thesaurus, Application Domain, Technology Topic, Popular Technical Reports.
© 2024 PatSnap. All rights reserved.Legal|Privacy policy|Modern Slavery Act Transparency Statement|Sitemap|About US| Contact US: help@patsnap.com