Smoke detection method and system based on foreground and background analysis and electronic equipment
A technology of foreground background and detection method, applied in the field of smoke detection, can solve the problems of false negatives and false positives, and achieve the effect of reducing false negatives and false positives, and improving sensitivity and accuracy.
- Summary
- Abstract
- Description
- Claims
- Application Information
AI Technical Summary
Problems solved by technology
Method used
Image
Examples
Embodiment 1
[0037] In this embodiment, deep learning is still used for smoke detection, but confrontation generation technology is added, including 3 sub-networks smoke detection network D, smoke removal network G, smoke analysis network S, and 4 difference functions smoke motion loss L S , background difference L B , scene restoration difference L R and background legacy L DS ,Such as figure 1 shown. Among them, the total loss of the network is to extract frame I from the video stream from the camera t , the foreground image is generated by the smoke detection network D Background image generation via smoke removal network G foreground image and background Image Synthesis of New Scene Images the foreground image of the current frame Generate smoke motion loss L through smoke analysis network S S .
[0038] Background image for the current frame Background image with previous frame The difference is Accumulate and leave L for the background B ,which is:
[0039] ...
Embodiment 2
[0050] This embodiment discloses a smoke detection network D, which uses a codec network to generate a foreground image from the current frame It The network parameter is θ D , this process can be described as:
[0051]
[0052] The encoder consists of four convolutional layers that transform the image It into high-dimensional features of size 1 / 24, 512 channels. The decoder uses 4 symmetric deconvolution layers to convert from high-dimensional features to the original size of the smoke channel image Each layer has a kernel size of 5, a stride of 2, and uses leaky ReLU and batch normalization operations. The corresponding layers of the decoder and the encoder are connected by a jump layer to preserve high-level information to ensure that the upsampled pixels can resolve the smoke with high quality. Network structure such as figure 2 As shown, the smoke is gradient, and the generated smoke channel image does not have a large gradient, so the smoke detection loss inclu...
Embodiment 3
[0056] This embodiment discloses a smoke removal network G, which uses a codec network to generate t generate background image The network parameter is θ G , the process is described as
[0057]
[0058] The encoder contains 8 convolutional layers to convert the input into a high-dimensional feature of 512 channels, and the decoder uses 8 symmetrical deconvolution layers to convert the high-dimensional feature into the background image of the original size If the encoder keeps reducing the resolution, and then the decoder keeps increasing the resolution, only in this way there will be problems of gradient disappearance and information imbalance of each layer. In fact, the background content of the surveillance camera rarely changes, and the jump connection can make this part of the information directly used for generation without going through the calculation process of compression and decompression to avoid the loss of information. Network structure such as image 3 ...
PUM
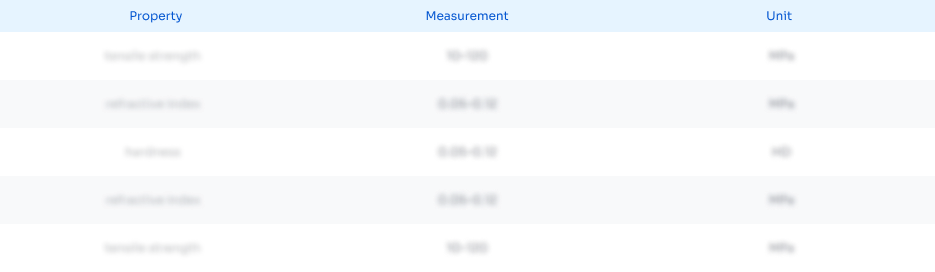
Abstract
Description
Claims
Application Information
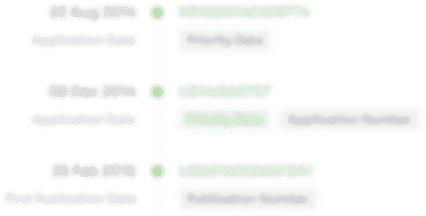
- Generate Ideas
- Intellectual Property
- Life Sciences
- Materials
- Tech Scout
- Unparalleled Data Quality
- Higher Quality Content
- 60% Fewer Hallucinations
Browse by: Latest US Patents, China's latest patents, Technical Efficacy Thesaurus, Application Domain, Technology Topic, Popular Technical Reports.
© 2025 PatSnap. All rights reserved.Legal|Privacy policy|Modern Slavery Act Transparency Statement|Sitemap|About US| Contact US: help@patsnap.com