Multi-joint robot control method based on adaptive neural network sliding mode control
A multi-joint robot and neural network control technology, applied in the direction of program control of manipulators, manipulators, manufacturing tools, etc., can solve problems such as difficult to establish multi-joint robot models
- Summary
- Abstract
- Description
- Claims
- Application Information
AI Technical Summary
Problems solved by technology
Method used
Image
Examples
Embodiment 1
[0063] see Figure 1-Figure 2 , a multi-joint robot control method based on adaptive neural network sliding mode control, the operation steps are as follows:
[0064] a. Construct the model of the multi-joint robot:
[0065] The dynamic model of the multi-joint robot is established by using the Lagrangian dynamic equation as follows:
[0066]
[0067] In the formula, is the inertia matrix of the articulated robot, Indicates the centrifugal force and Gothic force, is the gravity term, Indicates the system uncertainty caused by modeling errors, additional disturbances, etc., ΔD(q), ΔG(q) is the matrix D(q), respectively, Modeling error of G(q), τ d (t) is the additional disturbance force, are the rotation angle, angular velocity and angular acceleration of the joint, T(t) is the output torque, n is the number of joints; for a double-joint robot, n=2, the matrix D(q), and G(q) expressions are as follows:
[0068]
[0069]
[0070]
[0071] In the for...
Embodiment 2
[0111] This embodiment is basically the same as Embodiment 1, especially in that:
[0112] refer to Figure 1 to Figure 5 , a multi-joint robot control method based on an adaptive neural network sliding mode controller, the operation steps are as follows:
[0113] a. Model of multi-joint robot
[0114] According to the content of the above-mentioned content of the invention a, the dynamic model of the double-joint robot is as follows:
[0115]
[0116] In the formula, q=[q 1 q 2 ] T ,q 1 ,q 2 is the motion angle of the robot joint. m 1 =4.58kg, m 2 =6.52kg, l 1 = 0.3m, l 2 =0.35m, g=9.8m / s 2 , where Kg is mass in kilograms, m is length in meters, and s is time in seconds. The initial position is q(0)=[0.1 0.6] T , Interference is set to
[0117] b. Adaptive Neural Network Sliding Mode Control
[0118] According to the content of the above-mentioned content of the invention b, the multi-joint robot controller based on the adaptive neural network sliding ...
PUM
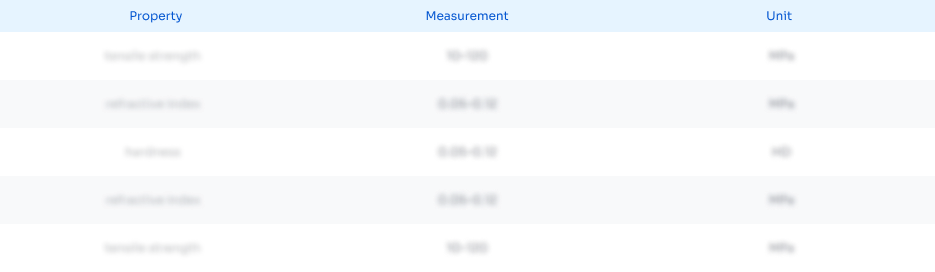
Abstract
Description
Claims
Application Information
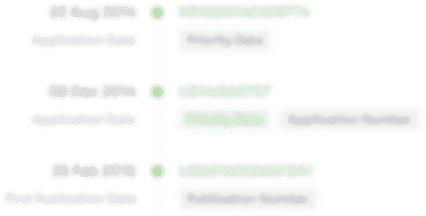
- R&D Engineer
- R&D Manager
- IP Professional
- Industry Leading Data Capabilities
- Powerful AI technology
- Patent DNA Extraction
Browse by: Latest US Patents, China's latest patents, Technical Efficacy Thesaurus, Application Domain, Technology Topic, Popular Technical Reports.
© 2024 PatSnap. All rights reserved.Legal|Privacy policy|Modern Slavery Act Transparency Statement|Sitemap|About US| Contact US: help@patsnap.com