Vision-based deep imitation reinforcement learning driving strategy training method
A technology of reinforcement learning and driving strategy, applied in the direction of neural learning methods, biological neural network models, neural architectures, etc., can solve problems such as inability to handle unknown scenarios, time-consuming, time-consuming training, etc., to improve the processing ability of unknown environments, The effect of ensuring comfort and safety and reducing learning costs
- Summary
- Abstract
- Description
- Claims
- Application Information
AI Technical Summary
Problems solved by technology
Method used
Image
Examples
Embodiment Construction
[0045] The present invention will be further described in detail below in conjunction with the accompanying drawings and specific embodiments: taking this as an example to further describe and illustrate the present application. Apparently, the described embodiments are only some of the embodiments of the present invention, not all of them.
[0046] This embodiment proposes a vision-based deep imitation reinforcement learning driving strategy training method, combining the advantages of imitation learning and deep reinforcement learning, obtaining initial driving strategy learning through imitation learning, and then solving the online driving strategy learning problem by deep reinforcement learning. The output of imitation learning is used as the input of deep reinforcement learning, which reduces the exploration space and improves the learning efficiency; at the same time, deep reinforcement learning solves the driving strategy learning of unknown environment, thereby improvi...
PUM
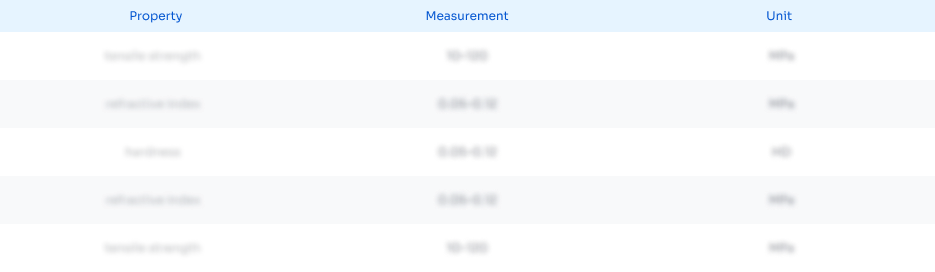
Abstract
Description
Claims
Application Information
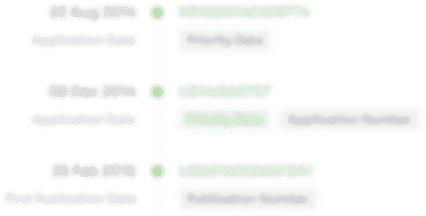
- R&D Engineer
- R&D Manager
- IP Professional
- Industry Leading Data Capabilities
- Powerful AI technology
- Patent DNA Extraction
Browse by: Latest US Patents, China's latest patents, Technical Efficacy Thesaurus, Application Domain, Technology Topic, Popular Technical Reports.
© 2024 PatSnap. All rights reserved.Legal|Privacy policy|Modern Slavery Act Transparency Statement|Sitemap|About US| Contact US: help@patsnap.com