Video super-resolution recovery method based on deep learning and adjacent frames
A super-resolution and deep learning technology, applied in the field of computer image processing, which can solve problems such as single features, no information learned, and no consideration of adjacent frame pictures.
- Summary
- Abstract
- Description
- Claims
- Application Information
AI Technical Summary
Problems solved by technology
Method used
Image
Examples
Embodiment 1
[0053] A video super-resolution restoration method based on deep learning and adjacent frames, comprising the following steps:
[0054] (1) Data preprocessing:
[0055] Preprocess the data set and divide the data set into training set and test set;
[0056] The data set is preprocessed, and the selected data set is the data set REDS for video super-resolution. The data set is divided into training set and test set; the training set includes 266 videos, and the test set includes 4 videos. Each of these videos has 100 frames. The low resolution and high resolution image resolutions are 320*180*3 and 1280*720*3 respectively. Among them, 320*180 and 1280*720 indicate the size of the image, and 3 indicates that the image is three channels.
[0057] (2) Data enhancement:
[0058] Crop the image into a small picture with a size of 64*64 to facilitate training. Randomly flip and rotate the image (0°, 90°, 180°, 270°) to increase the data.
[0059] (3) Data conversion:
[0060]...
Embodiment 2
[0066] According to a kind of video super-resolution recovery method based on deep learning and adjacent frames described in embodiment 1, its difference is:
[0067] The frame alignment module adopts full convolution, the convolution method is ordinary convolution and deformable convolution, and a pyramid cascade structure is used; the pyramid cascade structure includes three layers L1 layer, L2 layer, and L3 layer, which are processed by step (2) The feature map of L1 obtained by the ordinary convolution of the obtained low-scoring small picture, the feature map of L2 is obtained by down-sampling and convolution of the feature map of L1, and the feature map of L3 is obtained by down-sampling and convolution of the feature map of L2; the specific structure Such as figure 2 . That is, the frame alignment feature map output by the frame alignment module.
[0068] The reference frame, that is, the t-th frame image, and each of its adjacent frames, that is, the t+i-th frame im...
PUM
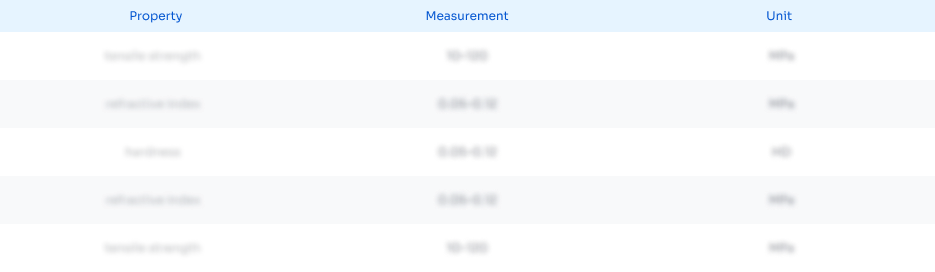
Abstract
Description
Claims
Application Information
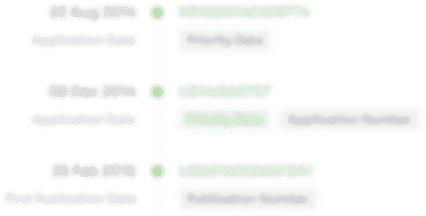
- R&D
- Intellectual Property
- Life Sciences
- Materials
- Tech Scout
- Unparalleled Data Quality
- Higher Quality Content
- 60% Fewer Hallucinations
Browse by: Latest US Patents, China's latest patents, Technical Efficacy Thesaurus, Application Domain, Technology Topic, Popular Technical Reports.
© 2025 PatSnap. All rights reserved.Legal|Privacy policy|Modern Slavery Act Transparency Statement|Sitemap|About US| Contact US: help@patsnap.com