Short-term load prediction method and system of LSTM neural network
A short-term load forecasting, neural network technology, applied in neural learning methods, biological neural network models, neural architectures, etc., can solve problems such as difficulty in predicting large-scale data, long computing time, and failure to take into account, and achieve accurate load forecasting. degree of deficiencies, avoid unfavorable influencing factors, and avoid the effect of false IMF
- Summary
- Abstract
- Description
- Claims
- Application Information
AI Technical Summary
Problems solved by technology
Method used
Image
Examples
Embodiment 1
[0099] Example 1, select the load data from June 16 to June 30, 2019 in a certain area, the sampling interval is 15 minutes, and a total of 1440 sampling points are used. The data of the previous 12 days are used as training samples, that is, the value of M is 3 , and the data of the following 3 days are used as test samples. In the VMD method, the value of K is 5, the value of the secondary penalty factor α is 1000, the value of the convergence criterion r is 10-6, and the initial center frequency w is 0. After optimization by the particle swarm optimization algorithm, the value of the parameter vector after LSTM network optimization is X opt =[2,50,0.65,16]. The LSTM model, EMD-LSTM model in the current commonly used methods and the load forecasting model based on the VMD method and LSTM neural network proposed in this study are compared, and the model is evaluated based on the Mean Absolute Percentage Error (MAPE). , the calculation formula of MAPE index is as follows:
...
PUM
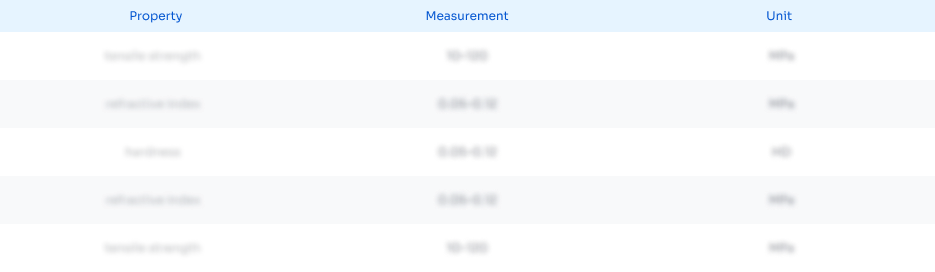
Abstract
Description
Claims
Application Information
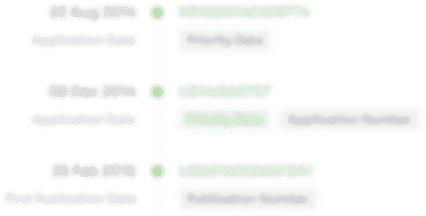
- R&D
- Intellectual Property
- Life Sciences
- Materials
- Tech Scout
- Unparalleled Data Quality
- Higher Quality Content
- 60% Fewer Hallucinations
Browse by: Latest US Patents, China's latest patents, Technical Efficacy Thesaurus, Application Domain, Technology Topic, Popular Technical Reports.
© 2025 PatSnap. All rights reserved.Legal|Privacy policy|Modern Slavery Act Transparency Statement|Sitemap|About US| Contact US: help@patsnap.com