Multi-auxiliary-domain information fusion cross-domain recommendation method and system
A recommendation method and auxiliary domain technology, applied in the direction of neural learning methods, digital data information retrieval, biological neural network models, etc., can solve the problems of easy loss of part of feature information, recommendation, etc., to alleviate data sparsity, performance optimization, recommendation list complete effect
- Summary
- Abstract
- Description
- Claims
- Application Information
AI Technical Summary
Problems solved by technology
Method used
Image
Examples
Example Embodiment
[0076] Example 1
[0077] Such as figure 2 As shown, a multi-auxiliary domain information fusion cross-domain recommendation method, including the following steps:
[0078] S1: Collect the data set and perform the data set, divide the data field processing, the domain C is the target domain, the domain B, the field M, and the domain E is an aided domain;
[0079] S2: Multiple auxiliary domains are used with Dctor models based on self-focus mechanism, namely the SA-Dctor model, and training, respectively, and obtain common user information matrices in the corresponding field and target domain, respectively.
[0080] S3: The plurality of common user information matrix inputs the self-focus mechanism module and splicing the fused vector to obtain a fusion matrix of score information and text information after multi-domain fusion;
[0081] S4: Enter the fusion matrix into a multi-layer perceived machine for nonlinear relationships between users and items;
[0082] S5: Enter the outpu...
Example Embodiment
[0086] Example 2
[0087] More specifically, for a common user information extraction section of a single model, use self-focus mechanism instead of the original poolization technology, the principle of self-focus mechanism image 3 As shown, Query and Key are hidden states of decoders and encoders, and Value is an embedding vector to extract information. Query and Key have generated corresponding weight A after a cost of calculating similarity, normalization, mask, and SoftMax. The obtained weight A is multiplied by the information vector value, which gives weights for each input vector according to the similarity. It will make it unforeseen or special. (Specifically, using max-pooling will remain in two fields, that is, the specialty but loss of generality. Use Average-Pool, simply retain the mean of both, that is, the general but lost specialty.)
[0088] More specifically, the DCTOR model (hereinafter referred to as the SA-Dctor Model) is based on self-focus mechanism. Figure 4...
Example Embodiment
[0146] Example 3
[0147] More specifically, the present invention is based on the integrated model of integrated model, and proposes a multi-auxiliary domain information fusion model based on self-focus mechanism and DCTOR, such as the N-SA-DCTOR model. Figure 10 Indicated. Assuming that the domain C is the target domain, the domain B, the field M, and the domain E are allocated. The first step, first use the auxiliary domain to use the Dctor model (hereinafter referred to as the SA-Dctor model) based on the self-focus mechanism, respectively, and generate a common user information vector D of the primary domain and each auxiliary domain, respectively. mc , D bc And D ec . In the second step, the plurality of common users are input to a layer of self-focus mechanism for weighting fusion, and the fused vector is condensed to obtain a multi-domain fusion vector U. The third step uses a fully connected multi-layer perception (MLP) to learn the nonlinear relationship between the use...
PUM
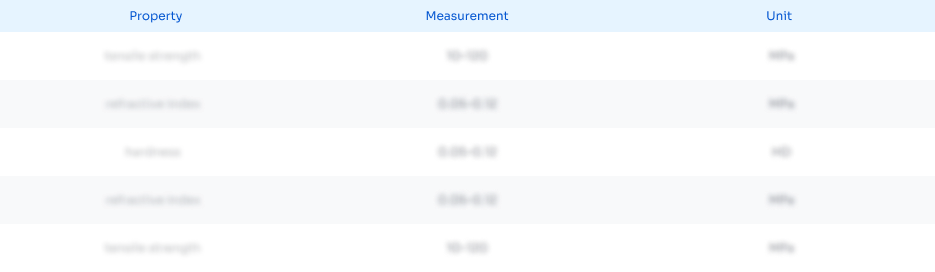
Abstract
Description
Claims
Application Information
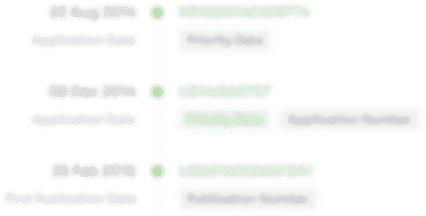
- R&D Engineer
- R&D Manager
- IP Professional
- Industry Leading Data Capabilities
- Powerful AI technology
- Patent DNA Extraction
Browse by: Latest US Patents, China's latest patents, Technical Efficacy Thesaurus, Application Domain, Technology Topic.
© 2024 PatSnap. All rights reserved.Legal|Privacy policy|Modern Slavery Act Transparency Statement|Sitemap