Traffic space-time sequence multi-step prediction method and system and storage medium
A multi-step forecasting and sequence technology, applied in forecasting, neural learning methods, biological neural network models, etc., can solve the problems of forecasting accuracy, difficult training, and affecting forecasting accuracy, and achieve the effect of improving forecasting accuracy
- Summary
- Abstract
- Description
- Claims
- Application Information
AI Technical Summary
Problems solved by technology
Method used
Image
Examples
Embodiment Construction
[0061] Since the raw data collected by the sensor or the smart terminal cannot be directly processed by the deep learning algorithm, the embodiment of the present invention first needs to preprocess the raw data to obtain grid data that can be processed by the deep learning algorithm. Some mathematical symbols are also involved in the algorithm. In order to facilitate the description, the definitions that need to be used in the proposed algorithm are first described. These definitions refer to the literature (X.Shi and D.-Y.Yeung, "Machine learning for spatiotemporalsequence forecasting" :A survey,”arXivPrepr.arXiv1808.06865,2018. and J.Zhang,Y.Zheng,and D.Qi,”Deep spatio-temporal residual networks for citywide crowdflows prediction,” in Thirty-First AAAI Conference on Artificial Intelligence, 2017.), further elaborate the process of preprocessing.
[0062] Define 1-1 (space division) grid(i,j). like image 3 As shown, let the coordinates of point A in the lower left corner ...
PUM
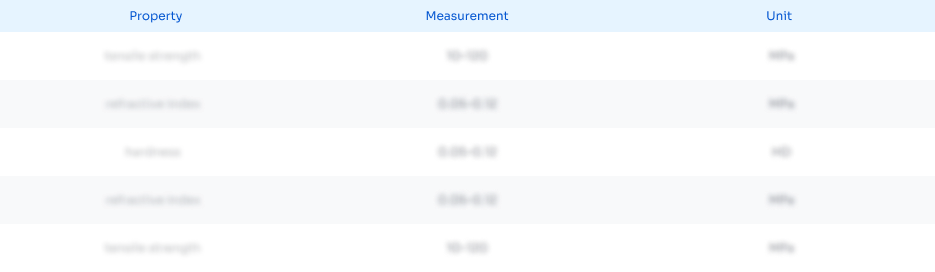
Abstract
Description
Claims
Application Information
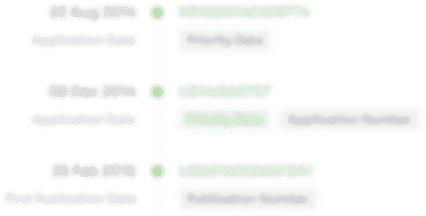
- R&D Engineer
- R&D Manager
- IP Professional
- Industry Leading Data Capabilities
- Powerful AI technology
- Patent DNA Extraction
Browse by: Latest US Patents, China's latest patents, Technical Efficacy Thesaurus, Application Domain, Technology Topic, Popular Technical Reports.
© 2024 PatSnap. All rights reserved.Legal|Privacy policy|Modern Slavery Act Transparency Statement|Sitemap|About US| Contact US: help@patsnap.com