Deep learning and transfer learning-based rock stratum structure intelligent detection and classification method
A technology of intelligent detection and classification method, which is applied in the field of intelligent detection and classification of rock strata structure based on deep learning and migration learning, and can solve the problem that there is no integrated software and hardware solution for intelligent detection and classification of rock stratum structure.
- Summary
- Abstract
- Description
- Claims
- Application Information
AI Technical Summary
Problems solved by technology
Method used
Image
Examples
Embodiment 1
[0070] 1. Analysis objectives, analysis content and key issues to be solved
[0071] Analysis goals:
[0072] Construct Inception-ResNet and YOLO perceptual neural network models based on existing borehole image data and acoustic logging data, normalize massive borehole image data, establish relatively complete training sets and test sets, and use them for network training and testing; For practical engineering applications, a pseudo-twinned convolutional neural network (PSCNN) with synchronous multi-input optical images and acoustic detection signals and a conditional generative adversarial network (CGAN) model based on multi-source and multi-scale real-time detection data are constructed; through deep learning and Transfer learning, to obtain a more adaptable and intelligent deep perception model, to solve the problem of intelligent identification and real-time classification of rock formation structures in measured holes. The main analysis objectives are as follows:
[00...
specific Embodiment approach 1
[0128] For the optical drilling image or acoustic image data collected by the drilling camera system, such as Figure 4 shown. The specific implementation route is as Figure 5 shown. use Figure 4 The labeled sub-module images are used to train and test the deep perceptual neural network based on Inception-ResNet and YOLO models. The tag data includes: clay, gravel, white dike, black hole, continuous structural surface, fracture zone, fault, marble, etc. After waiting for the network model training test to pass, it can be used to test the image characteristics of the remaining sub-modules in the borehole image: that is, re-input the borehole image data into the trained and tested deep perception network model, and the network model will then give the images that match The sub-module information of the label data feature, that is, output the label data in the image, such as clay, gravel, white vein, black hole, continuous structural surface, fracture zone, fault, marble, e...
specific Embodiment approach 2
[0130] For the optical borehole image and acoustic wave image data collected from the same borehole, the complementarity of optical image and acoustic wave data is used to complement each other's advantages. That is, the deep perception network model based on the pseudo twin convolutional neural network PSCNN and the conditional generative confrontation network CGAN model is used to conduct synchronous training and testing with labeled data. The optical drilling image and the acoustic wave image data are used as two synchronous input sources, and the same set of label data is the only output result. Especially when the output label data cannot be determined by the optical drilling image, further reference to the acoustic image data can further interpret and verify the authenticity of the final output label data. The specific implementation route is as Figure 8 shown. Among them, 6 is a double-input data source, 7 is a schematic diagram of the deep perception network structu...
PUM
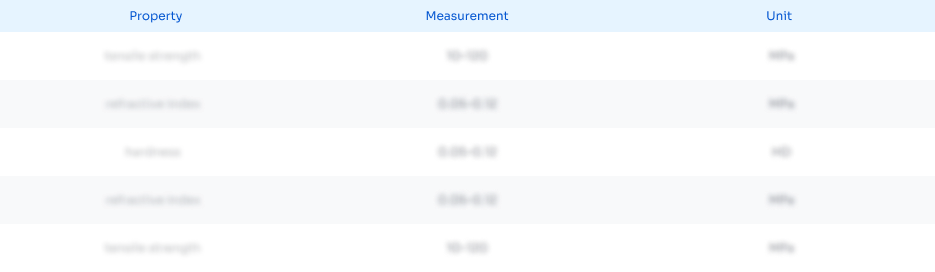
Abstract
Description
Claims
Application Information
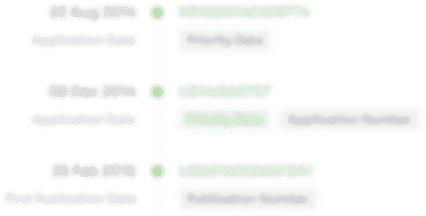
- R&D Engineer
- R&D Manager
- IP Professional
- Industry Leading Data Capabilities
- Powerful AI technology
- Patent DNA Extraction
Browse by: Latest US Patents, China's latest patents, Technical Efficacy Thesaurus, Application Domain, Technology Topic, Popular Technical Reports.
© 2024 PatSnap. All rights reserved.Legal|Privacy policy|Modern Slavery Act Transparency Statement|Sitemap|About US| Contact US: help@patsnap.com