Federal learning algorithm for bearing fault diagnosis
A technology of fault diagnosis and learning algorithm, applied in the field of Internet of Things, can solve the problems of limited effective information, cannot be deleted, poor scalability model, etc., to achieve the effect of improving real-time response, reducing training time, and reducing computing load
- Summary
- Abstract
- Description
- Claims
- Application Information
AI Technical Summary
Problems solved by technology
Method used
Image
Examples
Embodiment Construction
[0051] The technical solutions in the embodiments of the present invention will be described clearly and in detail below with reference to the drawings in the embodiments of the present invention. The described embodiments are only some of the embodiments of the invention.
[0052] The technical scheme that the present invention solves the problems of the technologies described above is:
[0053] figure 2 The target bearings of the Federal Bearing Fault Diagnosis System are shown. The type of bearing that needs to be diagnosed is deep groove ball bearing skf6205. Faulty bearings are machined by EDM. image 3 The overall framework of the system is shown, including two types of nodes: aggregation nodes and local nodes. Each local node is an edge server, which contains sensors for various components and the environment and a controller for controlling equipment. The edge server in the workshop aggregates the data of the sensor network to realize the processing of the underly...
PUM
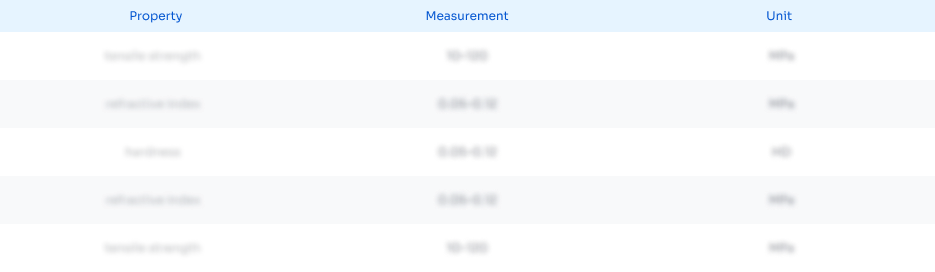
Abstract
Description
Claims
Application Information
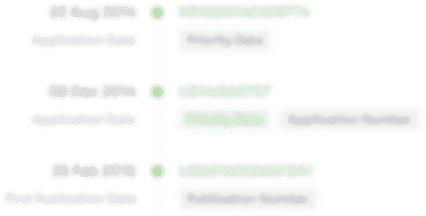
- R&D Engineer
- R&D Manager
- IP Professional
- Industry Leading Data Capabilities
- Powerful AI technology
- Patent DNA Extraction
Browse by: Latest US Patents, China's latest patents, Technical Efficacy Thesaurus, Application Domain, Technology Topic, Popular Technical Reports.
© 2024 PatSnap. All rights reserved.Legal|Privacy policy|Modern Slavery Act Transparency Statement|Sitemap|About US| Contact US: help@patsnap.com