Underground carry-scraper fault diagnosis method based on PSO-BP neural network
A PSO-BP, BP neural network technology, applied in neural learning methods, biological neural network models, mechanically driven excavators/dredgers, etc., can solve problems that are easy to fall into local optimal solutions, and fault diagnosis technology cannot timely feedback faults type and failure degree, to save a lot of time, improve diagnosis efficiency, and optimize the effect.
- Summary
- Abstract
- Description
- Claims
- Application Information
AI Technical Summary
Problems solved by technology
Method used
Image
Examples
Embodiment 1
[0118] The fault diagnosis method of the present invention will now be described by taking five types of common faults of underground scrapers as examples. The five types of faults selected in this embodiment are: engine coolant temperature is too high, piston cooling pressure is low, transmission oil leaks, diesel pollution leads to low traction and brake system pressure sensor failure. First extract the original operating data corresponding to these faults from the original database and dump them, then preprocess the dumped fault data according to the process described in step 2, and then process the data set according to step 3 After calibration, it is substituted into the Relief algorithm for feature selection and filtering, in which the number of sample sampling m is set to 200, and the threshold is set to 11, that is, the output is ranked as the top 11 attributes. The feature parameter data set filtered and selected by the Relief algorithm includes 11-dimensional feature...
PUM
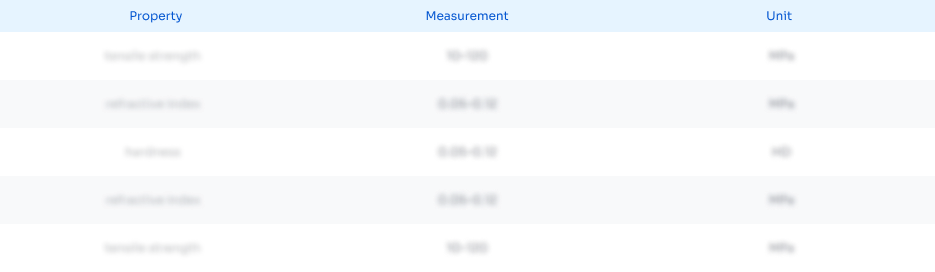
Abstract
Description
Claims
Application Information
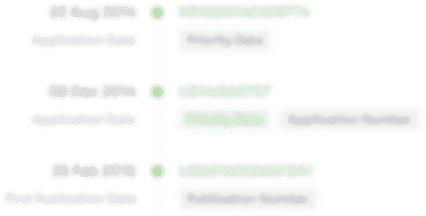
- R&D Engineer
- R&D Manager
- IP Professional
- Industry Leading Data Capabilities
- Powerful AI technology
- Patent DNA Extraction
Browse by: Latest US Patents, China's latest patents, Technical Efficacy Thesaurus, Application Domain, Technology Topic.
© 2024 PatSnap. All rights reserved.Legal|Privacy policy|Modern Slavery Act Transparency Statement|Sitemap