Channel prediction method combining deep learning and basis extension model
A base extension model and channel prediction technology, applied in the field of channel prediction, can solve the problems of increased prediction complexity, low prediction accuracy, and high computational complexity, and achieve the effects of reduced computational complexity, high prediction accuracy, and high prediction performance
- Summary
- Abstract
- Description
- Claims
- Application Information
AI Technical Summary
Problems solved by technology
Method used
Image
Examples
Embodiment Construction
[0044] The present invention is further described below in conjunction with accompanying drawing:
[0045] Such as Figure 1-5 A channel prediction method of a joint deep learning and base extension model is shown, including the following steps:
[0046] Step 1: Obtain the correlation matrix of the channel according to the channel information at historical moments
[0047] In the formula, H m =[H 1,m ,...H k,m ,...,H K,m ], where H k,m is the frequency-domain channel coefficient of the k-th subcarrier of the m-th symbol obtained by using least-squares estimation and linear interpolation for the received pilot signal, and N is the length of the OFDM symbol;
[0048] Step 2: Decompose the eigenvalue of the channel correlation matrix to obtain the optimal basis function
[0049] B m =U m (:,1:Q)
[0050] In the formula, U m is the eigenvector matrix corresponding to the eigenvalues arranged in descending order, U m (:,1:Q) is U m A vector composed of the first Q ...
PUM
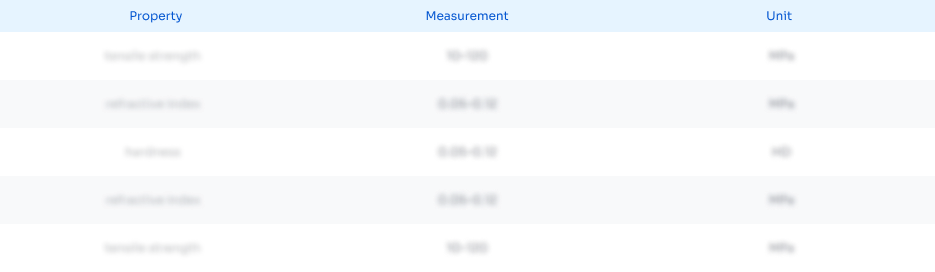
Abstract
Description
Claims
Application Information
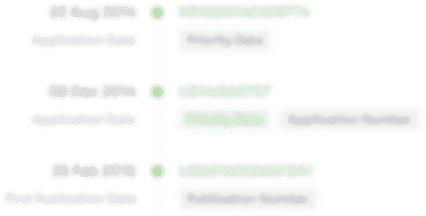
- R&D Engineer
- R&D Manager
- IP Professional
- Industry Leading Data Capabilities
- Powerful AI technology
- Patent DNA Extraction
Browse by: Latest US Patents, China's latest patents, Technical Efficacy Thesaurus, Application Domain, Technology Topic, Popular Technical Reports.
© 2024 PatSnap. All rights reserved.Legal|Privacy policy|Modern Slavery Act Transparency Statement|Sitemap|About US| Contact US: help@patsnap.com