High-resolution image rape extraction method based on deep learning algorithm
A technology of deep learning and extraction methods, applied in the field of remote sensing image processing, can solve the problems that remote sensing images are difficult to meet the resolution requirements of fragmented rapeseed, limit the generalization and generalization ability of a single model, and limit the extraction accuracy of classification analysis methods, etc. Achieve the effect of improving accuracy and model generalization ability
- Summary
- Abstract
- Description
- Claims
- Application Information
AI Technical Summary
Problems solved by technology
Method used
Image
Examples
Embodiment Construction
[0091] Embodiments of the technical solutions of the present invention will be described in detail below in conjunction with the accompanying drawings. The following examples are only used to illustrate the technical solutions of the present invention more clearly, and therefore are only examples, rather than limiting the protection scope of the present invention.
[0092] The implementation of this case is as follows:
[0093] Step 1: Data collection and preprocessing:
[0094] (1) Gaofen-2 image acquisition: Acquire Gaofen-2 images during the flowering period of rapeseed (end of February to mid-April);
[0095] (2) Gaofen-2 image data preprocessing: The acquired Gaofen-2 images are preprocessed sequentially by radiometric calibration, orthorectification, image registration, atmospheric correction, and image fusion. The specific steps are:
[0096] a. Radiometric calibration: convert the DN value of Gaofen-2 image into apparent radiance (L λ ):
[0097] L λ = Gain λ ×DN+O...
PUM
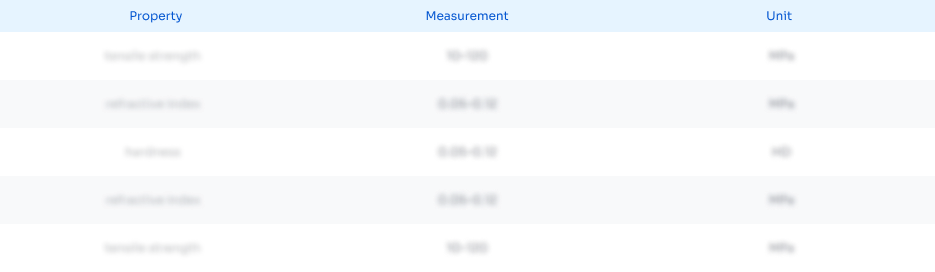
Abstract
Description
Claims
Application Information
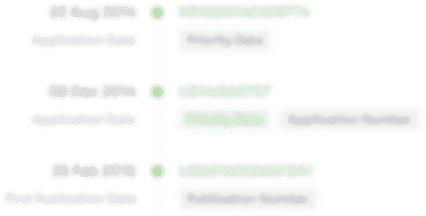
- R&D Engineer
- R&D Manager
- IP Professional
- Industry Leading Data Capabilities
- Powerful AI technology
- Patent DNA Extraction
Browse by: Latest US Patents, China's latest patents, Technical Efficacy Thesaurus, Application Domain, Technology Topic, Popular Technical Reports.
© 2024 PatSnap. All rights reserved.Legal|Privacy policy|Modern Slavery Act Transparency Statement|Sitemap|About US| Contact US: help@patsnap.com