Heart MR image interpolation method and system based on causal relationship
A causal relationship and image technology, applied in the field of image processing, can solve problems such as signal loss, blurred image quality, missing, etc., to achieve the effect of improving accuracy and fast operation speed
- Summary
- Abstract
- Description
- Claims
- Application Information
AI Technical Summary
Problems solved by technology
Method used
Image
Examples
Embodiment 1
[0050] like Figure 1-5 As shown, Embodiment 1 of the present disclosure provides a causal-based cardiac MR image interpolation method, including the following process:
[0051] Obtain the cardiac MR image to be processed and perform preprocessing;
[0052]Key point extraction is performed on the preprocessed current frame cardiac MR image to obtain a sequence of key point coordinates based on time series;
[0053] According to the obtained key point coordinate sequence, combined with the graph neural network, the interactive causal relationship between each pair of key points is obtained;
[0054] According to the obtained interaction causality, the coordinates of the key points in the next frame image are obtained;
[0055] According to the coordinates of the key points in the next frame image, the missing images in the heart MR sequence are obtained.
[0056] Specifically, include the following:
[0057] Step (1): Receive the public data of cardiac MR short-axis sequenc...
Embodiment 2
[0083] Embodiment 2 of the present disclosure provides a cardiac MR image interpolation system based on causality, including:
[0084] The image acquisition module is configured to: acquire the cardiac MR image to be processed and perform preprocessing;
[0085] The key point extraction module is configured to: perform key point extraction on the preprocessed current frame heart MR image, and obtain a sequence of key point coordinates based on time series;
[0086] The causal relationship determination module is configured to: obtain the interactive causal relationship between each pair of key points according to the obtained key point coordinate sequence, combined with the graph neural network;
[0087] The key point prediction module is configured to: obtain the coordinates of the key point in the next frame image according to the obtained interactive causal relationship;
[0088] The image interpolation module is configured to: obtain the missing images in the heart MR seq...
Embodiment 3
[0091] Embodiment 3 of the present disclosure provides a computer-readable storage medium, on which a program is stored. When the program is executed by a processor, the method of cardiac MR image interpolation based on causality as described in Embodiment 1 of the present disclosure is implemented. step.
PUM
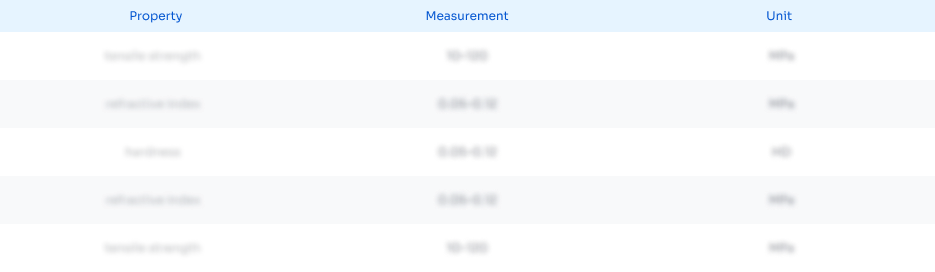
Abstract
Description
Claims
Application Information
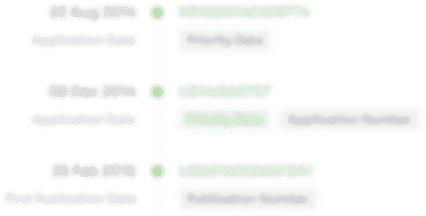
- R&D Engineer
- R&D Manager
- IP Professional
- Industry Leading Data Capabilities
- Powerful AI technology
- Patent DNA Extraction
Browse by: Latest US Patents, China's latest patents, Technical Efficacy Thesaurus, Application Domain, Technology Topic, Popular Technical Reports.
© 2024 PatSnap. All rights reserved.Legal|Privacy policy|Modern Slavery Act Transparency Statement|Sitemap|About US| Contact US: help@patsnap.com