Atmospheric turbulence phase space-time estimation algorithm
An atmospheric turbulence, space-time technology, applied in the field of adaptive optics, can solve the problems that cannot meet the real-time performance and accuracy of adaptive optics system phase measurement, cannot meet the real-time performance of adaptive optics system phase measurement, and the time-series image features cannot be very good. It can meet the real-time requirements, the calculation speed is fast, and the structure is simple.
- Summary
- Abstract
- Description
- Claims
- Application Information
AI Technical Summary
Problems solved by technology
Method used
Image
Examples
Embodiment Construction
[0059] The present invention will be further described below in conjunction with the accompanying drawings and embodiments, and the present invention includes but not limited to the following embodiments.
[0060] Embodiment 1 of the present invention provides a space-time prediction algorithm for atmospheric turbulence phase based on deep learning, such as figure 1 As shown, it includes two stages of training and estimation, which are divided into the following steps:
[0061] a. The steps in the training phase are:
[0062] S1. Use the phase acquisition device to obtain the time-series atmospheric turbulence phase map introduced by atmospheric turbulence. The atmospheric turbulence phase map is marked as Pi in turn, where i=1, 2, 3, 4...T, T is the maximum of the obtained time-series phase information number of frames. The time interval between adjacent frames is equal;
[0063] S2. Process T frames of atmospheric turbulence phase map into N pieces of equal-length atmosph...
PUM
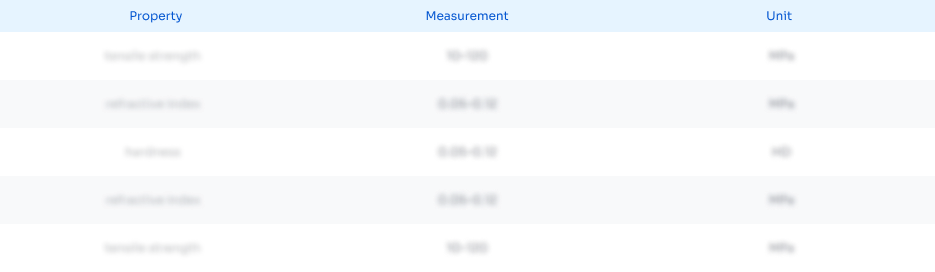
Abstract
Description
Claims
Application Information
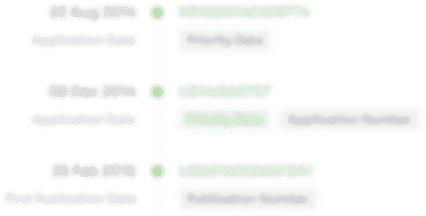
- Generate Ideas
- Intellectual Property
- Life Sciences
- Materials
- Tech Scout
- Unparalleled Data Quality
- Higher Quality Content
- 60% Fewer Hallucinations
Browse by: Latest US Patents, China's latest patents, Technical Efficacy Thesaurus, Application Domain, Technology Topic, Popular Technical Reports.
© 2025 PatSnap. All rights reserved.Legal|Privacy policy|Modern Slavery Act Transparency Statement|Sitemap|About US| Contact US: help@patsnap.com