Pipeline defect intelligent detection method based on image processing and deep learning, and application thereof
A deep learning and intelligent detection technology, applied in the field of Internet of Things and artificial intelligence, can solve problems such as difficult to distinguish steel pipe defects, inability to detect corrosive defects, poor detection and training effects, etc.
- Summary
- Abstract
- Description
- Claims
- Application Information
AI Technical Summary
Problems solved by technology
Method used
Image
Examples
Embodiment 1
[0112] The defect detection model is obtained by the following process:
[0113] data collection
[0114] In the present invention, the data consists of two parts: self-made data set and NEU public data set. The self-made data set comes from a video data set of an oil mining site, and some of its video images are as follows: Figure 4 shown. Since the collected on-site data set mainly comes from short-term video, the data has certain regularity. In order to avoid this problem, the collected video is extracted by frame to obtain image data.
[0115] Because the invention is used to detect whether there are defects in oil pipelines, in order to achieve better detection results, NEU public data sets are added to supplement data diversity. The original label classification of the public data set is: rolling scale (RS), plaque (Pa), cracking (Cr), pitting surface (PS), inclusions (Is) and scratches (Sc), a total of 6 categories Different types of surface defects.
[0116] data...
PUM
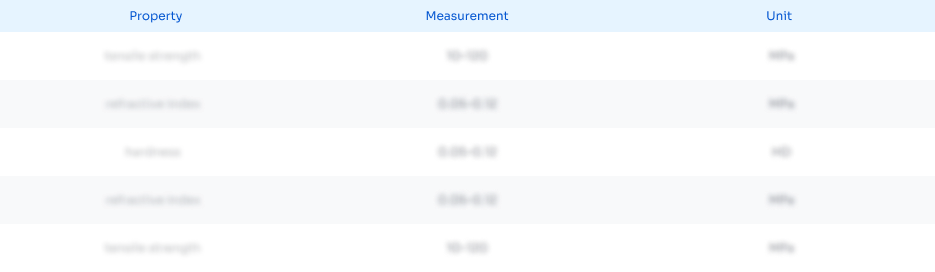
Abstract
Description
Claims
Application Information
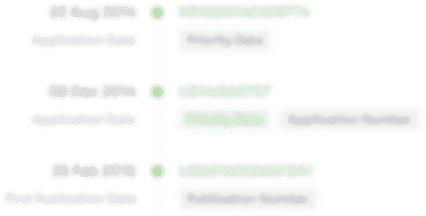
- R&D
- Intellectual Property
- Life Sciences
- Materials
- Tech Scout
- Unparalleled Data Quality
- Higher Quality Content
- 60% Fewer Hallucinations
Browse by: Latest US Patents, China's latest patents, Technical Efficacy Thesaurus, Application Domain, Technology Topic, Popular Technical Reports.
© 2025 PatSnap. All rights reserved.Legal|Privacy policy|Modern Slavery Act Transparency Statement|Sitemap|About US| Contact US: help@patsnap.com