Diabetic retinopathy image classification method based on improved ResNeSt convolutional neural network model
A convolutional neural network and retinopathy technology, applied in the field of medical image processing, can solve the problems of uneven diagnosis level, time-consuming and labor-intensive efficiency, misdiagnosis, etc., achieve good classification results, improve accuracy, and reduce parameters.
- Summary
- Abstract
- Description
- Claims
- Application Information
AI Technical Summary
Problems solved by technology
Method used
Image
Examples
Embodiment Construction
[0046] Below in conjunction with accompanying drawing and embodiment the present invention is described in further detail:
[0047] A kind of diabetic retinopathy image classification method based on improved ResNeSt convolutional neural network model, specifically comprises the following steps:
[0048] Step 1. Obtain medical images of diabetic retinopathy from the hospital;
[0049] Step 2. Preprocess the collected medical images first, and then ask professional ophthalmologists to manually label the lesions in the medical images to form a dataset with labeling information required for training the ResNeSt convolutional neural network classification model. Divide the dataset with labeled information into three parts: training set, validation set and test set;
[0050] Step 3. Build the deep learning server platform required for the experiment, and then write the python code to prepare the model;
[0051] Step 4. By introducing two lightweight and efficient convolution operat...
PUM
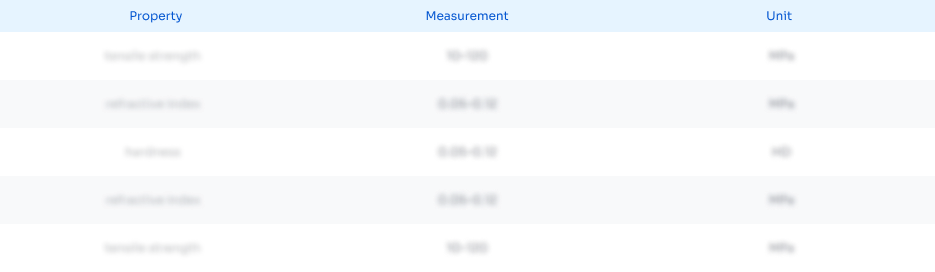
Abstract
Description
Claims
Application Information
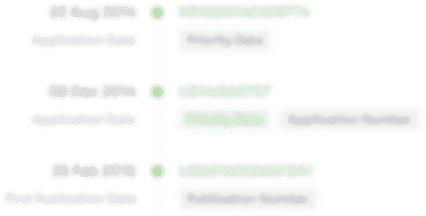
- R&D Engineer
- R&D Manager
- IP Professional
- Industry Leading Data Capabilities
- Powerful AI technology
- Patent DNA Extraction
Browse by: Latest US Patents, China's latest patents, Technical Efficacy Thesaurus, Application Domain, Technology Topic.
© 2024 PatSnap. All rights reserved.Legal|Privacy policy|Modern Slavery Act Transparency Statement|Sitemap