Real-time fault diagnosis method and system based on sequential random forest
A random forest, real-time fault technology, applied in random CAD, computer parts, character and pattern recognition, etc., can solve the problems of high missed diagnosis rate and misdiagnosis rate, failure to realize fault diagnosis, etc., to alleviate the missed diagnosis rate and misdiagnosis rate bias. High, real-time adaptive multi-sample fault diagnosis effect
- Summary
- Abstract
- Description
- Claims
- Application Information
AI Technical Summary
Problems solved by technology
Method used
Image
Examples
Embodiment 1
[0023] figure 1 It is a flow chart of a sequential random forest-based real-time fault diagnosis method provided according to an embodiment of the present invention, and the method is applied to fault detection of a nonlinear system. Such as figure 1 As shown, the method specifically includes the following steps:
[0024] Step S102, acquiring the residual signal to be measured of the nonlinear system to be diagnosed.
[0025] Step S104, extracting the time-frequency feature vector of the residual signal to be measured based on wavelet packet decomposition.
[0026] In step S106, the time-frequency feature vector is substituted into the trained random forest fault separator, and a sequential probability ratio test is performed on the nonlinear system to be diagnosed to obtain target fault information. Wherein, the target fault information includes target fault type and target fault occurrence time.
[0027] In step S108, the time-frequency feature vector and the target faul...
Embodiment 2
[0116] image 3 is a schematic diagram of a sequential random forest-based real-time fault diagnosis system provided according to an embodiment of the present invention. Such as image 3 As shown, the system includes: an acquisition module 10 , an extraction module 20 , a fault separation module 30 and a fault identification module 40 .
[0117] Specifically, the acquiring module 10 is configured to acquire the residual signal to be measured of the nonlinear system to be diagnosed.
[0118] Optionally, the obtaining module 10 is also used to: establish a nominal model of the nonlinear system to be diagnosed, and obtain a nominal system residual signal of the nominal model; obtain an observation residual signal of the nonlinear system to be diagnosed; The system residual signal and the observation residual signal are used to obtain the residual signal to be measured.
[0119] Specifically, the residual signal to be tested is determined by the following formula: e l =e s -g...
PUM
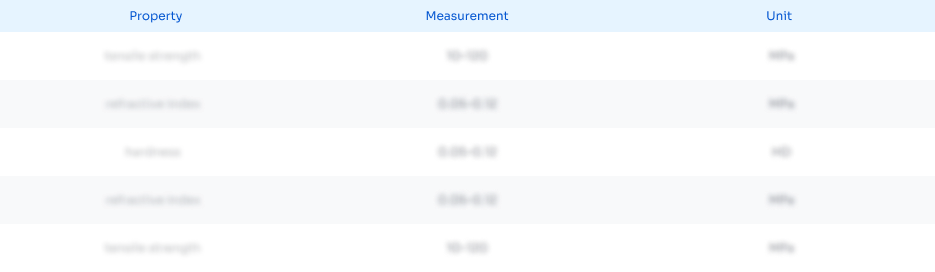
Abstract
Description
Claims
Application Information
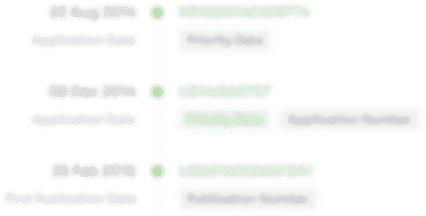
- R&D
- Intellectual Property
- Life Sciences
- Materials
- Tech Scout
- Unparalleled Data Quality
- Higher Quality Content
- 60% Fewer Hallucinations
Browse by: Latest US Patents, China's latest patents, Technical Efficacy Thesaurus, Application Domain, Technology Topic, Popular Technical Reports.
© 2025 PatSnap. All rights reserved.Legal|Privacy policy|Modern Slavery Act Transparency Statement|Sitemap|About US| Contact US: help@patsnap.com