Tunnel surrounding rock pressure prediction method for slurry balance shield, equipment and storage medium
A technology of surrounding rock pressure and mud-water balance, applied in neural learning methods, neural architecture, design optimization/simulation, etc., can solve problems such as surface collapse, noise tunnel face pressure fluctuation, and excavation face mud pressure prediction, etc. Predicting and reducing the probability of construction accidents and subsequent use of tunnels, and the effect of eliminating noise interference
- Summary
- Abstract
- Description
- Claims
- Application Information
AI Technical Summary
Problems solved by technology
Method used
Image
Examples
Embodiment 1
[0047] Example 1 illustrates a flowchart of a proposed method for predicting highly fluctuating tunnel parameters. refer to figure 1 , S represents the time series of PLC data in a ring. First, the denoising method based on VMD-DFA (Variational Mode Decomposition-Detrended Fluctuation Analysis) is used to obtain the denoising signal of the PLC data time series. BLIMFs (Band-limited Intrinsic ModeFunctions, intrinsic mode components of limited bandwidth) are expressed as u1, u2,...uk,uk+1,...uk_optimal. u1, u2, ... uk are considered as denoised signals, the parameter k is determined by the scaling exponent, and other components of BLIMFs are considered as noise. The determination of K_optimal will be given in the next embodiment. Cross-correlation analysis (Canonical Correlation Analysis, CCA) is performed on the denoised signal to distinguish the trend component and the fluctuation component. Two predictors based on the strength and fluctuation of LSTM (Long Short-Term Mem...
Embodiment 2
[0048] Example 2 describes how the denoising method works. Regarding the variational mode decomposition (VMD) part: the VMD algorithm is used to decompose the PLC data time series S(t) in the ring into discrete mode numbers uk, k=1, 2, ... K_optimal. Each uk mode has a narrowband center frequency wk. In order to determine uk and wk, the VMD algorithm needs to solve the constrained variational problem (formula (1)):
[0049]
[0050] where t is the time script, δ is the Dirac function, and * denotes convolution. {uk} and {wk} denote the obtained modes and center frequencies, respectively. Application of the quadratic penalty α and the Lagrangian multiplier λ transforms Equation (1) into Equation (2):
[0051]
[0052] Equation (2) can be solved by the Alternating Direction Method of Multipliers (ADMMs) of the multiplier algorithm. The solution can be expressed as equation (3).
[0053]
[0054] This problem can be solved in the frequency domain with the help of th...
Embodiment 3
[0067] Example 3 describes a PLC data prediction model based on coupled CCA-LSTM. About CCA: Cross-correlation analysis (CCA) is used to study the relationship between denoised PLC data and its decomposed components. The cross-correlation function and uj of noisy time series, 1≤j≤k, are defined as formula (11):
[0068]
[0069] Sdenoised and uj are the mean values of each time series, and N is the length of the time series. T is the time log, indicating that the feature that was noisy at time T appears in uj at time t+T. Based on δj, PLC data after denoising, S trend and S denoised The trend and volatility components will be determined.
[0070] About LTSM: As a recurrent neural network (RNN), LSTM network uses a gate mechanism to overcome the vanishing gradient problem caused by the recursive structure of RNN. figure 2 The detailed structure of the gate is shown. The forward calculation method is shown in formula group (13). Three gates are used in the LSTM unit...
PUM
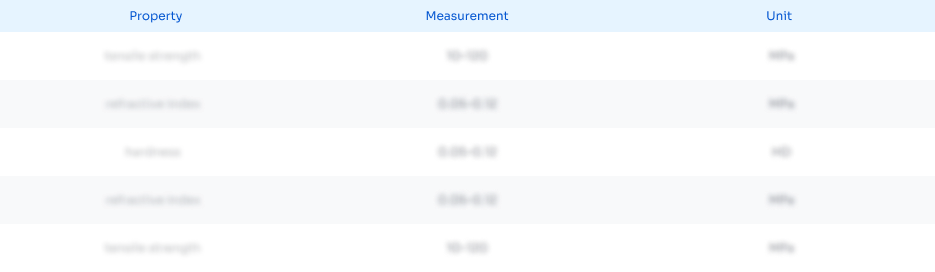
Abstract
Description
Claims
Application Information
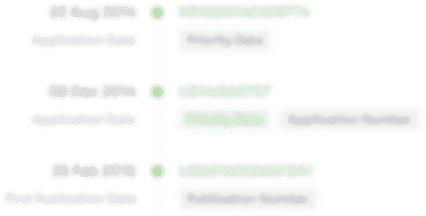
- R&D Engineer
- R&D Manager
- IP Professional
- Industry Leading Data Capabilities
- Powerful AI technology
- Patent DNA Extraction
Browse by: Latest US Patents, China's latest patents, Technical Efficacy Thesaurus, Application Domain, Technology Topic, Popular Technical Reports.
© 2024 PatSnap. All rights reserved.Legal|Privacy policy|Modern Slavery Act Transparency Statement|Sitemap|About US| Contact US: help@patsnap.com