Complex industrial process fault prediction method based on RF noise reduction self-encoding information reconstruction and time convolution network
- Summary
- Abstract
- Description
- Claims
- Application Information
AI Technical Summary
Problems solved by technology
Method used
Image
Examples
Embodiment Construction
[0077] According to the actual chemical reaction process, American Eastman Chemical Company has developed an open and challenging chemical model simulation platform - Tennessee Eastman (TE) simulation platform. The TE chemical process is a prototype of an actual process flow. The whole process includes 5 main operating units, namely reactor, condenser, cycle compressor, gas-liquid separator and product desorption tower. The data generated by it have time-varying and strong coupling and nonlinear features, widely used for testing control and fault diagnosis models of complex industrial processes.
[0078] There are 41 measured variables and 12 manipulated variables in the TE process, and 21 fault types are set. The 12th manipulated variable XMV(12) remains unchanged throughout the process and is not used as input data. Select 52 variables [XMEAS(1), ..., XMEAS(41), XMV(1), ..., XMV(11)] as the original observation signal vector at a specific moment. In the simulation experime...
PUM
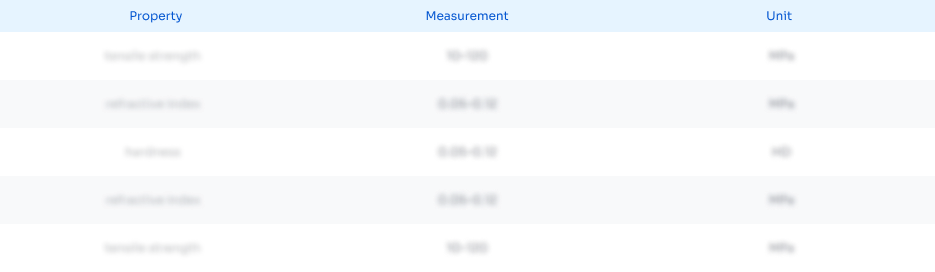
Abstract
Description
Claims
Application Information
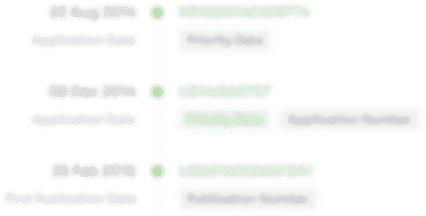
- R&D Engineer
- R&D Manager
- IP Professional
- Industry Leading Data Capabilities
- Powerful AI technology
- Patent DNA Extraction
Browse by: Latest US Patents, China's latest patents, Technical Efficacy Thesaurus, Application Domain, Technology Topic, Popular Technical Reports.
© 2024 PatSnap. All rights reserved.Legal|Privacy policy|Modern Slavery Act Transparency Statement|Sitemap|About US| Contact US: help@patsnap.com