Community discovery method based on nonlinear non-negative matrix factorization
A technology of non-negative matrix decomposition and community discovery, applied in the field of community discovery based on nonlinear non-negative matrix decomposition, it can solve problems such as lack of learning ability and inability to improve community discovery performance, achieving the effect of strong expressive ability and improved performance
- Summary
- Abstract
- Description
- Claims
- Application Information
AI Technical Summary
Problems solved by technology
Method used
Image
Examples
Embodiment 1
[0043] Such as figure 1 with figure 2 As shown, a community discovery method based on nonlinear non-negative matrix factorization, including the following steps:
[0044] S1: Formalize the social network and generate an undirected graph;
[0045] S2: According to the undirected graph, introduce the graph convolutional network GCN and NMF model to construct a unified loss function;
[0046] S3: Optimizing the unified loss function to obtain a local optimal solution;
[0047] S4: Extract the community discovery results according to the local optimal solution.
[0048] In the above scheme, the graph convolution network GCN and NMF model are introduced to construct a unified loss function, and joint training is performed to endow NMF with the learning ability of nonlinear features, so as to improve the performance of the NMF-based community discovery method and have strong expressive ability.
[0049] Preferably, in step S1, the undirected graph is G=(V,E), where V={v 1 ,......
Embodiment 2
[0071] figure 2 It is the overall model framework of the method, which includes an input layer, a graph convolution layer, and an output layer. The input layer uses the adjacency matrix and identity matrix of the graph as input, and the graph convolution layer uses a 2-layer graph convolution network. The activation function is ReLU , the output layer is responsible for outputting the graph node representation Z normalized by the Softmax function, and used to reconstruct the adjacency matrix of the graph. The whole model framework is trained by constructing graph reconstruction and NMF joint loss function.
specific Embodiment approach
[0074] Step 1: Formally represent the social network. Formalize the social network example as G=(V,E), where V=(v 0 ,v 1 ,v 2 ,v 3 ,v 4 ,v 5 ), E={e 01 ,e 02 ,e 12 ,e 23 ,e 34 ,e 35 ,e 45}, n=6. The corresponding adjacency matrix A and identity matrix I are:
[0075]
[0076]
[0077] Step 2: Construct the unified loss function of GCN and NMF: Here α is set to 1, and the number of communities k is set to 2.
[0078] Step 3: Optimize the solution of the unified loss function. Solve by iteratively updating the rules as follows:
[0079]
[0080] Step 4: Get community discovery results. Set the number of iterations t=10, and the result of H obtained after iteration convergence is:
[0081]
[0082] According to H, the two communities to be discovered can be directly judged c 0 and c 1 members, for example, for user node v 0 , its corresponding community affiliation strength distribution vector in H is [0.63,0.01], 0.63>0.01, so c0=c 0 ∪{v 0}, s...
PUM
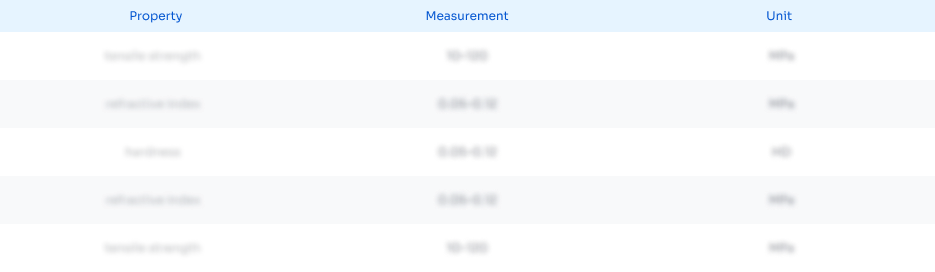
Abstract
Description
Claims
Application Information
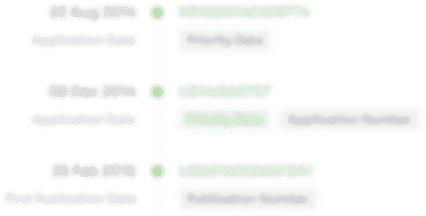
- R&D Engineer
- R&D Manager
- IP Professional
- Industry Leading Data Capabilities
- Powerful AI technology
- Patent DNA Extraction
Browse by: Latest US Patents, China's latest patents, Technical Efficacy Thesaurus, Application Domain, Technology Topic, Popular Technical Reports.
© 2024 PatSnap. All rights reserved.Legal|Privacy policy|Modern Slavery Act Transparency Statement|Sitemap|About US| Contact US: help@patsnap.com