Epileptic seizure prediction method through electroencephalogram signal on the basis of multi-scale convolution and self-attention network
A technology of EEG signals and epileptic seizures, applied in diagnostic recording/measurement, medical science, sensors, etc., can solve a large number of problems such as manual extraction, low error prediction rate, and lack of universality, so as to increase adaptability, Solve the effect of information forgetting and increase width
- Summary
- Abstract
- Description
- Claims
- Application Information
AI Technical Summary
Problems solved by technology
Method used
Image
Examples
Embodiment Construction
[0026]The technical solution adopted in the present invention is a method for predicting epileptic seizures based on a multi-scale convolution self-attention network of original EEG signals. The network is used for epilepsy detection research on single-channel EEG data, using one-dimensional convolution for feature extraction and dimensionality reduction for EEG signals, and then using Bi-directional Long-Short-Term Memory (BiLSTM) for Classification. BiLSTM is a combination of forward LSTM and backward LSTM. When processing data with a time series relationship, it is often better than unidirectional LSTM, because the information conveyed by BiLSTM needs to be determined by several previous inputs and subsequent inputs. Based on this network, combined with the Inception idea and the Transformer self-attention coding layer, this study proposes a Multi-Scale CSANet structure to realize the use of multi-channel EEG signals to predict epileptic seizures.
[0027] The specific imp...
PUM
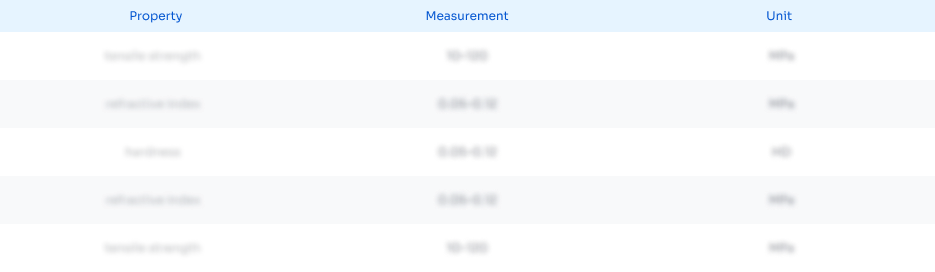
Abstract
Description
Claims
Application Information
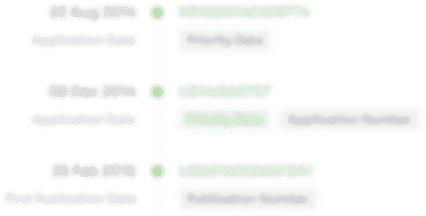
- R&D
- Intellectual Property
- Life Sciences
- Materials
- Tech Scout
- Unparalleled Data Quality
- Higher Quality Content
- 60% Fewer Hallucinations
Browse by: Latest US Patents, China's latest patents, Technical Efficacy Thesaurus, Application Domain, Technology Topic, Popular Technical Reports.
© 2025 PatSnap. All rights reserved.Legal|Privacy policy|Modern Slavery Act Transparency Statement|Sitemap|About US| Contact US: help@patsnap.com