Brain disease classification method based on 3D attention convolution and self-supervised learning
A supervised learning and disease classification technology, applied in the field of brain science research, can solve problems such as high feature dimension, high-dimensional data mining, and inability to reflect the spatial relationship of voxel data, and achieve the effect of reasonable and reliable methods and improved performance
- Summary
- Abstract
- Description
- Claims
- Application Information
AI Technical Summary
Problems solved by technology
Method used
Image
Examples
Embodiment Construction
[0060] Take the real fMRI data set as an example below to illustrate the specific implementation steps of the present invention:
[0061] Step (1) Obtain resting state fMRI data and preprocess:
[0062] Step (1.1) Resting-state fMRI data acquisition: Autism spectrum disorder (ASD) data was acquired from ABIDE (Autism Brain Imaging DataExchange, http: / / fcon_1000.projects.nitrc.org / indi / abide / ) for analysis; ABIDE-I contains rs-fMRI (Resting-state functional magnetic resonance imaging) data of 1112 subjects from 17 different sites; the quality of these data was assessed based on the results of visual inspection by three human experts, by incomplete Brain coverage, high motor peaks, ghosting, and other scanner artifacts generated 871 subjects from an initial sample of 1112 subjects, including 468 normal controls and 403 ASD; ABIDE-II collected The data of 1114 subjects from 17 different sites, 518 subjects were selected as the experimental data, including 265 people in the norma...
PUM
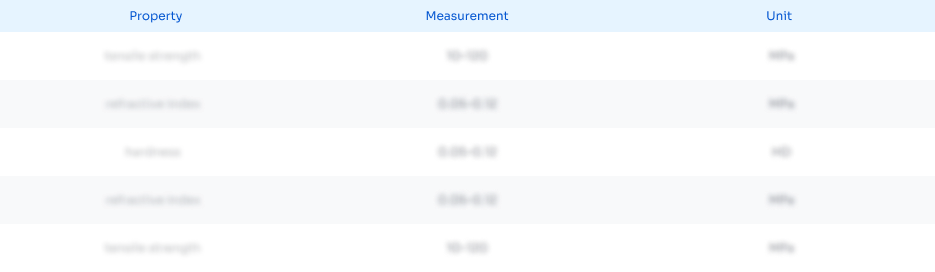
Abstract
Description
Claims
Application Information
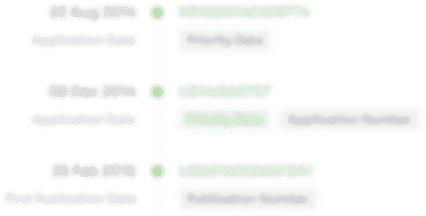
- R&D Engineer
- R&D Manager
- IP Professional
- Industry Leading Data Capabilities
- Powerful AI technology
- Patent DNA Extraction
Browse by: Latest US Patents, China's latest patents, Technical Efficacy Thesaurus, Application Domain, Technology Topic, Popular Technical Reports.
© 2024 PatSnap. All rights reserved.Legal|Privacy policy|Modern Slavery Act Transparency Statement|Sitemap|About US| Contact US: help@patsnap.com