Laser radar target detection method and device based on deep learning
A target detection and lidar technology, which is applied in the field of lidar target detection based on deep learning, can solve the problems of large amount of calculation, can not meet the deployment of automatic driving, and is not suitable for the field of automatic driving, so as to improve the upper limit of network performance and reduce the point Cloud information loss, the effect of satisfying real-time detection
- Summary
- Abstract
- Description
- Claims
- Application Information
AI Technical Summary
Problems solved by technology
Method used
Image
Examples
Embodiment 1
[0045] figure 1 A schematic flow chart of the deep learning-based laser radar target detection method provided in Embodiment 1 of the present invention, as shown in figure 1 As shown, the method includes the following steps:
[0046] Step 110, acquiring a laser point cloud; the laser point cloud includes multiple points, and each point includes three-dimensional coordinates in the first coordinate system.
[0047] Specifically, in the field of autonomous driving, for example, a laser radar is installed on an autonomous driving vehicle, and the laser radar can obtain laser point clouds in real time. The laser point cloud is a series of disordered spatial coordinate points. Three-dimensional coordinates refer to the position and height of each point in the laser point cloud, which can be represented by (x, y, h), where x represents the horizontal axis coordinate, y represents the vertical axis coordinate, and h represents the height. The first coordinate system may be a lidar ...
Embodiment 2
[0076] Figure 4 It is a schematic structural diagram of a laser radar target detection device based on deep learning provided by Embodiment 2 of the present invention. Such as image 3 As shown, the deep learning-based laser radar target detection device includes: an acquisition module 410 , a grid determination module 420 , a first learning module 430 , a second learning module 440 and a target detection frame determination module 450 .
[0077] The obtaining 410 module is used to obtain the laser point cloud; the laser point cloud includes a plurality of points, and each point includes three-dimensional coordinates in the first coordinate system;
[0078] The grid determination module 420 is used to determine the effective grid in the sub-grid after projecting the laser point cloud to a plurality of sub-grids of the plane grid, and determine the grid attribute of the effective grid; the grid attribute includes each effective The three-dimensional coordinates of the points...
Embodiment 3
[0089] Embodiment 3 of the present invention provides a chip system, including a processor, a coupling between the processor and a memory, the memory stores program instructions, and when the program instructions stored in the memory are executed by the processor, any one of the methods provided in Embodiment 1 can be realized. LiDAR object detection method.
PUM
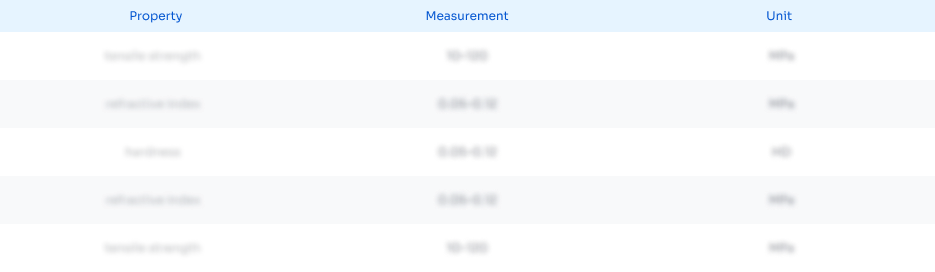
Abstract
Description
Claims
Application Information
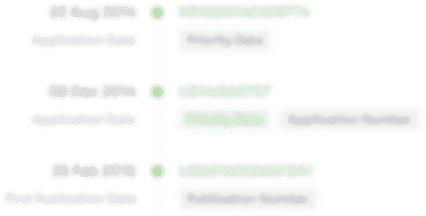
- R&D Engineer
- R&D Manager
- IP Professional
- Industry Leading Data Capabilities
- Powerful AI technology
- Patent DNA Extraction
Browse by: Latest US Patents, China's latest patents, Technical Efficacy Thesaurus, Application Domain, Technology Topic.
© 2024 PatSnap. All rights reserved.Legal|Privacy policy|Modern Slavery Act Transparency Statement|Sitemap