Lung CT image segmentation device based on spatial neighborhood analysis
A technology of neighborhood analysis and CT images, applied in the field of image processing, can solve the problems of not fully considering the three-dimensional spatial information of the lesion area, poor interpretability, difficult clinical practice, etc., to achieve low overall calculation, ensure accuracy, and improve segmentation The effect of precision
- Summary
- Abstract
- Description
- Claims
- Application Information
AI Technical Summary
Problems solved by technology
Method used
Image
Examples
Embodiment Construction
[0055] In order to make the structure and advantages of the present application clearer, the structure of the present application will be further described below with reference to the accompanying drawings.
[0056] combined with Figure 1 to Figure 5 , a lung CT image segmentation device based on spatial neighborhood analysis proposed in the embodiment of the present application includes an image preprocessing module, a spatial neighborhood feature identification module, a self-attention feature decoding module, and a multi-view region calibration module, wherein: The image preprocessing module normalizes the CT value under the lung window to the image pixel value according to the original lung CT image file, calculates the lung parenchyma foreground area mask corresponding to each layer, and combines the single foreground area layer and its front and rear neighborhood maps. The layers are combined into a set of neighborhood sequences for the subsequent feature extraction pro...
PUM
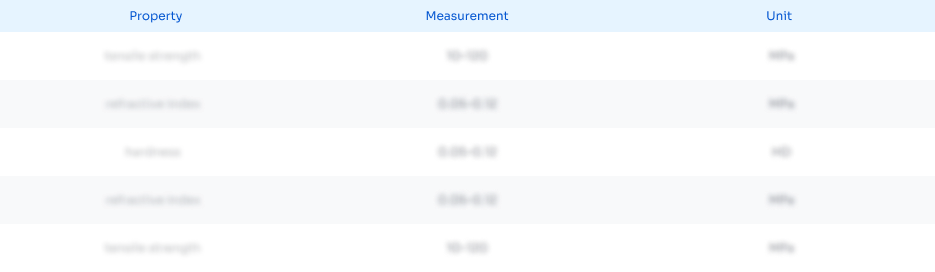
Abstract
Description
Claims
Application Information
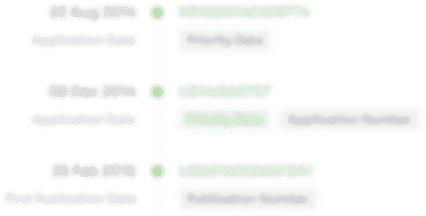
- R&D
- Intellectual Property
- Life Sciences
- Materials
- Tech Scout
- Unparalleled Data Quality
- Higher Quality Content
- 60% Fewer Hallucinations
Browse by: Latest US Patents, China's latest patents, Technical Efficacy Thesaurus, Application Domain, Technology Topic, Popular Technical Reports.
© 2025 PatSnap. All rights reserved.Legal|Privacy policy|Modern Slavery Act Transparency Statement|Sitemap|About US| Contact US: help@patsnap.com