Jinji network self-strengthening image voice deep learning model
A deep learning and self-reinforcing technology, applied in the field of image speech deep learning, can solve the problems of poor generalization ability, inability to transform, and unsatisfactory accuracy of local estimators, etc., to achieve enhanced self-learning ability, improved system stability, Effects with reasonable computational complexity
- Summary
- Abstract
- Description
- Claims
- Application Information
AI Technical Summary
Problems solved by technology
Method used
Image
Examples
Embodiment Construction
[0123] In order to make the purpose, features, advantages, and innovations of the present application more obvious and easy to understand and easy to implement, the specific embodiments are described in detail below with reference to the accompanying drawings. Those skilled in the art can make similar promotions without departing from the connotation of the present application, so the present application is not limited by the specific embodiments disclosed below.
[0124] Deep learning based on convolutional neural networks, including network structure, basic operations and training techniques. Although the network is becoming more and more efficient, the architecture design is more complex, requires a lot of professional knowledge and experience, and consumes a lot of time and cost. The design of the current neural network is still a big problem.
[0125] Current CNN architectures are mainly handcrafted through experiments or modified from a few existing networks, which requi...
PUM
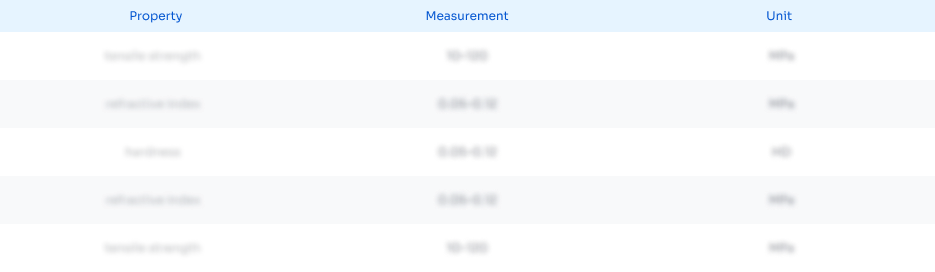
Abstract
Description
Claims
Application Information
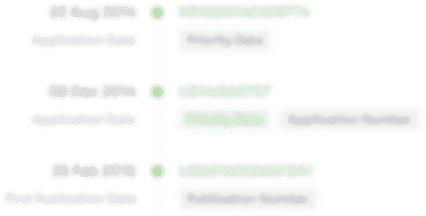
- Generate Ideas
- Intellectual Property
- Life Sciences
- Materials
- Tech Scout
- Unparalleled Data Quality
- Higher Quality Content
- 60% Fewer Hallucinations
Browse by: Latest US Patents, China's latest patents, Technical Efficacy Thesaurus, Application Domain, Technology Topic, Popular Technical Reports.
© 2025 PatSnap. All rights reserved.Legal|Privacy policy|Modern Slavery Act Transparency Statement|Sitemap|About US| Contact US: help@patsnap.com