Vehicle reinforcement learning motion planning method based on driving risk analysis
A motion planning and reinforcement learning technology, applied in two-dimensional position/channel control and other directions, can solve the problems of expensive trial and error training, difficult model convergence, lack of interpretability of model output actions, etc., to reduce the incidence of dangerous actions , the model has strong self-learning ability, and the effect of improving generalization ability and interpretability
- Summary
- Abstract
- Description
- Claims
- Application Information
AI Technical Summary
Problems solved by technology
Method used
Image
Examples
Embodiment Construction
[0064] Exemplary embodiments of the present disclosure will be described in more detail below with reference to the accompanying drawings. While exemplary embodiments of the present disclosure are shown in the drawings, it should be understood that the present disclosure may be embodied in various forms and should not be limited by the embodiments set forth herein. Rather, these embodiments are provided so that the present disclosure will be more thoroughly understood, and will fully convey the scope of the present disclosure to those skilled in the art. It should be noted that, unless otherwise specified, the technical or scientific terms used in the present invention should have the usual meanings understood by those skilled in the art to which the present invention belongs.
[0065] figure 1 A flow chart of a vehicle reinforcement learning motion planning method based on driving risk analysis provided according to an embodiment of the present invention. figure 2 The flow...
PUM
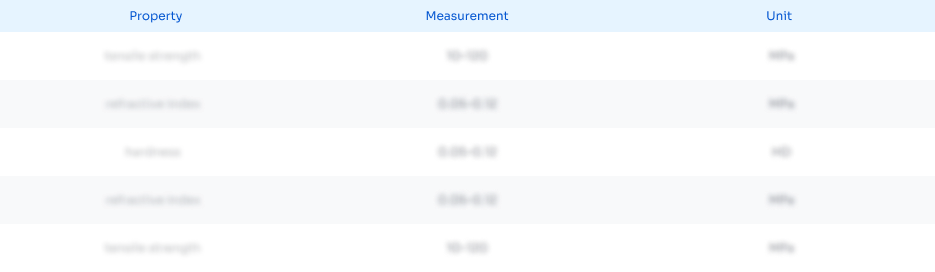
Abstract
Description
Claims
Application Information
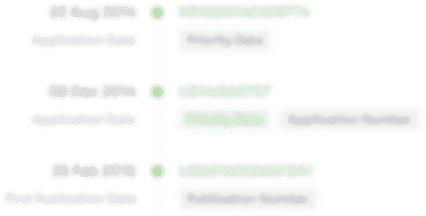
- R&D Engineer
- R&D Manager
- IP Professional
- Industry Leading Data Capabilities
- Powerful AI technology
- Patent DNA Extraction
Browse by: Latest US Patents, China's latest patents, Technical Efficacy Thesaurus, Application Domain, Technology Topic, Popular Technical Reports.
© 2024 PatSnap. All rights reserved.Legal|Privacy policy|Modern Slavery Act Transparency Statement|Sitemap|About US| Contact US: help@patsnap.com