Lithium ion battery residual life online prediction method based on deep learning algorithm
A lithium-ion battery and deep learning technology, which is applied in the field of online prediction of the remaining life of lithium-ion batteries based on deep learning algorithms, can solve problems such as reduced prediction accuracy, uncontrollable capacity regeneration, and difficult realization of charging and discharging processes, etc., to reduce computing power. cost, improving robustness and universality, and avoiding dependencies
- Summary
- Abstract
- Description
- Claims
- Application Information
AI Technical Summary
Problems solved by technology
Method used
Image
Examples
Embodiment 1
[0045] This embodiment is used to illustrate the method for online prediction of the remaining life of a battery disclosed in the present invention.
[0046] Step 1: The battery data set comes from the Center for Advanced Life Cycle Engineering of the University of Maryland. The battery is a 4.2V lithium cobalt oxide-graphite system lithium-ion battery (rated capacity: 1100mAh), and the charge-discharge cycle is performed at 0.5C / 1C (charge cut-off current is 0.05C). Select CS2-36, CS2-37 and CS2-38 as offline battery datasets, and CS2-35 as online battery. The voltage interval of all the complete discharge curves obtained was determined to be 2.710V-4.181V, and the voltage interval between each point on the discharge curve was set to 0.01V. Then fix the number of points n 1 A window of 30 slides within its voltage interval and is divided into discharge curve segments with a voltage interval of 0.30V. Each segment contains sampled signals (voltage and capacity signals) for ...
Embodiment 2
[0053] This embodiment is used to illustrate the method for online prediction of the remaining life of a battery disclosed in the present invention.
[0054] Step 1: The battery data set comes from the Center for Advanced Life Cycle Engineering of the University of Maryland. The battery is a 4.2V lithium cobalt oxide-graphite system lithium-ion battery (rated capacity: 1350mAh), and the charge-discharge cycle is performed at 0.5C / 1C (charge cut-off current is 0.05C). Select CX2-34, CX2-36 and CX2-37 as offline battery datasets, and CX2-38 as online battery. The voltage interval of all the complete discharge curves obtained is determined to be 2.700V-4.040V, and the voltage interval between each point on the discharge curve is set to 0.01V. Then fix the number of points n 1 A window of 30 slides within its voltage interval and is divided into discharge curve segments with a voltage interval of 0.30V. Each segment contains sampled signals (voltage and capacity signals) for th...
PUM
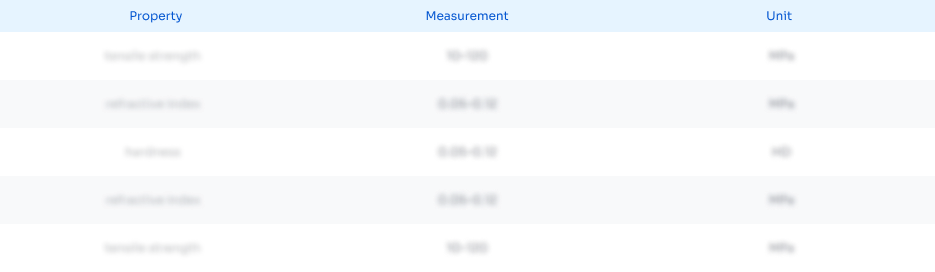
Abstract
Description
Claims
Application Information
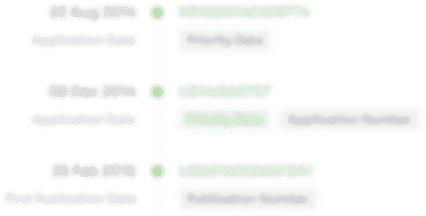
- R&D
- Intellectual Property
- Life Sciences
- Materials
- Tech Scout
- Unparalleled Data Quality
- Higher Quality Content
- 60% Fewer Hallucinations
Browse by: Latest US Patents, China's latest patents, Technical Efficacy Thesaurus, Application Domain, Technology Topic, Popular Technical Reports.
© 2025 PatSnap. All rights reserved.Legal|Privacy policy|Modern Slavery Act Transparency Statement|Sitemap|About US| Contact US: help@patsnap.com