Rolling bearing residual life prediction method based on LSTM and TDNN
A rolling bearing and life prediction technology, applied in prediction, neural learning methods, character and pattern recognition, etc., can solve the problems of neglecting diversity, poor method portability, lack of evaluation of conservative prediction or radical prediction, etc., to ensure prediction accuracy, Avoid the effect of sudden failure
- Summary
- Abstract
- Description
- Claims
- Application Information
AI Technical Summary
Problems solved by technology
Method used
Image
Examples
Embodiment 1
[0088] A method for predicting the remaining life of a rolling bearing based on LSTM and TDNN of the present invention is further described below with reference to the data of specific embodiments.
[0089] The simulation environment and parameters of this embodiment are selected as follows:
[0090] Simulation environment
[0091] Model: Intel(R) Core(TM) i3-9100 CPU@3.60GHz 3.60GHz;
[0092] Operating system: Windows 10 Professional;
[0093] Software: Matlab R2020a.
[0094] parameter settings
[0095] The original data of this example are taken from the accelerated life test of XJTU-SY rolling bearing jointly carried out by the team of Professor Lei Yaguo, School of Mechanical Engineering, Xi'an Jiaotong University and Zhejiang Changxing Shengyang Technology Co., Ltd., see Wang et al, IEEE Transactions on Reliability for details. , 2018, 69(1):401-412.
[0096]Taking working condition 1 in the above literature as an example, the detailed description of the experimenta...
PUM
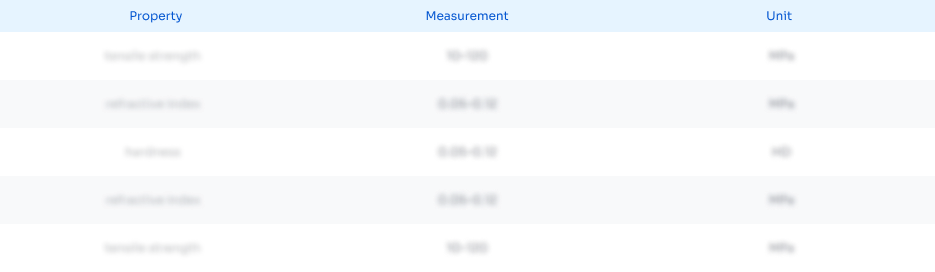
Abstract
Description
Claims
Application Information
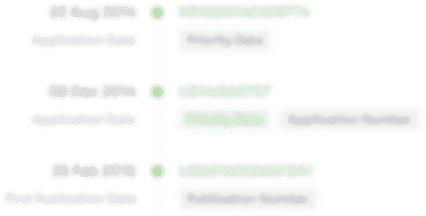
- R&D Engineer
- R&D Manager
- IP Professional
- Industry Leading Data Capabilities
- Powerful AI technology
- Patent DNA Extraction
Browse by: Latest US Patents, China's latest patents, Technical Efficacy Thesaurus, Application Domain, Technology Topic, Popular Technical Reports.
© 2024 PatSnap. All rights reserved.Legal|Privacy policy|Modern Slavery Act Transparency Statement|Sitemap|About US| Contact US: help@patsnap.com